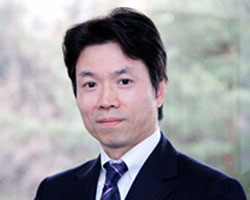 |
Naonori Ueda
Director, PhD
NTT CS-Labs.
Guest Professor of Nara Advanced
Institute of Science and Technology
Guest Professor of National Institute of Informatics
|

Biography
Naonori Ueda received the B.S., M.S., and Ph D degrees in Communication
Engineering from Osaka University, Osaka, Japan, in 1982, 1984, and 1992,
respectively. In 1984, he joined the Electrical Communication Laboratories,
NTT, Japan, where he was engaged in research on image processing, pattern
recognition, and computer vision. In 1991, he joined the NTT Communication
Science Laboratories, where he has invented a significant learning principle
for optimal vector quantizer design and has developed some novel learning
algorithms including deterministic annealing EM (DAEM) algorithm, ensemble
learning, the split and merge EM (SMEM) algorithm, semi-supervised learning,
variational Bayesian model search algorithm for mixture models and its
application to speech recognition, and probabilistic generative models
for multi-labeled text in WWW. His current research interests include parametric
and non-parametric Bayesian approach to machine learning, pattern recognition,
data mining, signal processing, and cyber-physical systems. From 1993 to
1994, he was a visiting scholar at Purdue University, West Lafayette, USA.
Currently, he is a director of NTT Communication Science Laboratories.
He is an associate editor of Neurocomputing and Journal of Neural Networks,
and is a member of the Institute of Electronics, Information, and Communication
Engineers (IEICE), and IEEE.
Awards
- Best Paper Award, ICONIP (International Conference on Neural Information Processing), 2009
- Best Paper Award, IPS (Information Processing Society), 2009
- Best Paper Award, Funai Foundation Award, 2007
- Telecommunication Advanced Foundation Award, 2005
- Yamashita SIG Research Award, IPS (Information Processing Society), 2005
- Paper Award, Funai Foundation Award, 2005
- Research Award, Japanese Society for Artificial Intelligence, 2005
- Meritorious Award, IEICE (Institute of Electronics, Information, and Communication
Engineers), 2004
- Best Paper Award, Funai Foundation Award, 2004
- Best Paper Award, IEICE (Institute of Electronics, Information, and Communication
Engineers), 2004
- Research Award (Japanese Neural Network Society (JNNS)), 2003
- Best Paper Award, IEICE (Institute of Electronics, Information, and Communication
Engineers), 2000
- Telecommunication Advanced Foundation Award, 1997
- Research Award, JNNS (Japanese Neural Network Society), 1995

Professional Activities
- Program committee,@Technical Committee, MPS, IPS 2006-March 2010.
* IPS : Information Processing Society
* MPS: Mathematical Modeling and Problem Solving
- Associate Editor, Neurocomputing Journal, 2004-present.
- Associate Editor, Neural Networks Journal, 2003-present.
- Part-time Researcher, RIKEN, The Institute of Physical and Chemical Research,
2003-present.
- Visiting Collaborated Research, Hinton Lab., University of College London,
UK, April 1999, August 2000.
- Visiting Collaborated Research, Hinton Lab., University of Toronto, CANADA, October 1997.
- Visiting Scholar, Purdue University, USA, 1993-1994.
- Senior Program Committee, ICML, 2007.
* ICML: International Conference on Machine Learning
- General Conference Board, JNNS, 2001.
- Board Member, JNNS, 2000-2001.
* JNNS: Japanese Neural Network Society
- General Chair, IBIS, 2003.
- Program Commitee, IBIS Workshop, 2000.
* IBIS: Information-Based Induction Science
- Fellow, IEICE, 2009
- General Chair, IBIS Technical Group, IEICE, 2003-2004.
- Editor in Chief, IEICE Transactions on Infomation and System (Special Issue
on IBIS), 2001.
- Paper Editorial Committee Member, IEICE, 1999-2002.
- Special Issue, Editorial board, IEICE, 1998, 2002-2003.
- Paper Review Member, IEICE, 1996-present.
- Editorial board, IEICE, 1996-1998.
* IEICE: The institute of Electronics, Information,and Communication Engineers
- Paper Review Member, NIPS, 1999-2002. 2005-present
* NIPS: Neural Information Processing Systems
- Program Committee, IEEE NNSP, 2001-2002.
* NNSP: Neural Networks for Signal Processing
- Paper Review Member, IEE, 1997-2000.
* IEE: The institute of Electrical Engineers
- Commitee Member, AVIRG, 1992-1993.
* AVIRG: Audio and Visual Information Research Group

Invited Academic Talks
- "Introduction to Nonparametric Bayesian Models," IPSJ CVIM/IEICE
PRMU, March 2009
- "Nonparametric Bayesian Learning," NHK Science and Technical
Research Labs, August 2007
- "Inference methods for Nonparametric Bayes models, Workshop on Bayesian Inference," (The Institute of Statistical Mathematics), August. 2007.
- "Multimedia Signal Processing," Osaka University, May 2007.
- "Nonparametric Bayesian Theory and its Application to Data Mining,"
SIG-DMSM, July 2006.
- "Mathematical MOodeling for Multiple Topics," Information ProcessingSociety
of Japan (IPSJ), Yamashita SIG Award Memorial Lecture, March 2006
- "Ensemble Learning," IEICE PRMU, September 2004.
- "New Development of Text Modeling," NLP, March 2003.
- "Variational Bayes/Parametric Mixture Models," ATR Spoken Language Translation Research Laboratories, July 2002.
- "Ensemble Learning," The Institute of Systems, Control, and Information Engineers, May 2002.
- "New development of the EM algorithm. -Variational Bayes- ,"
Meeting of Technical Group on Neural Computation, IEICE, January 2002.
- "Theory and applications of Variatiaonal Bayes," The Institute
of Statistical Mathematics, February 2001.
- "New Development of the EM Algorithm," Japanese Society of Computational
Statistics, October 2000.
- "The Front of Statistical Learning Theory," Kyoto University,
April 2000.
- "Optimal Model Search based on Bayesian Approach," Biometric
Analysis Dept., SHIONOGI & CO., LTD, March 2000.
- "Latent Variable Models and Probabilistic
Dimensionality Reduction," The Institute of Statistical Mathematics,
September 1998.
- "A Feature Extraction Method Based on Latent Variable
Models," Meeting of Technical Group on Pattern Recognition and Media
Understanding, IEICE, June 1998.
- "Probabilistic Neural Networks Based on Latent Variable Models," Yukawa Institute
for Theoretical Physics, Kyoto University, January 1998.
- "Mathematical Morphology," Meeting of Technical Group on
Precision Engineering, August 1996.
- "EM Algorithm," Bio-infomatics Research Meeting, October 1995.
- "Deterministic Annealing EM Algorithm," ATR Interpreting Telecommunications Research Laboratories, May 1995.
- "Deterministic Annealing EM Algorithm," GA Research Meeting, April 1995.
- "Ensemble Learning," Osaka University, January 1995.

Career
- Part-time Lecturer, (Tokyo University, Bayesian Learning Theory), 2007
- Guest Professor, (Nara Advanced Institute of Science and Technology (NAIST)), 2004-present.
- Guest Associate Professor, (Nara Advanced Institute of Science and Technology
(NAIST)), 1998-2004
- Part-time Lecturer, (Osaka University), 2003-present.
- Part-time Lecturer, (Kyoto University, Pattern Recognition and Bayesian
Learning), 2003-present.
- Part-time Lecturer, (Waseda University, Statistical Learning Theory), 2003.
- Part-time Lecturer, (Okayama University, Statistical Pattern Recognition), 1999.
- Part-time Lecturer, (Nagoya Institute of Technology, Electrical and Computer
Enginnering), 2002.
- Part-time Lecturer, (Tsukuba University, Statistical Learning Theory), 1999-2002.

Publications
Refereed Journal Papers
- K. Ishiguro, T. Iwata, and N. Ueda,"Dynamic infinite relational model for time-dependent relational data
analysis",Journal of Information Processing Society of Japan, Vol. 3, No.1, 1-12, 2010 (in Japanese)
- Tomoharu Iwata, Toshiyuki Tanaka, Takeshi Yamada, Naonori Ueda,"Model
Learning when Distributions Differ over Time,"Transactions of IEICEJ,
Vol.J92-D, No.3, 361-370, 2009 (in Japanese)
- Tomoharu Iwata, Takeshi Yamada, Naonori Ueda,"Visualizing Documents
based on Topic Models,"Journal of Information Processing Society of
Japan, Vol.50, No.6,1649-1659, 2009 (in Japanese)
- Kawamae, N., Sakano, H., Yamada, T., and Ueda, N.,"Collaborative filtering focusing on the dynamics and precedence of user
preference",Transactions of IEICEJ, (D-II), Vol. J92-DII, No.6, pp. 767-776, 2009, (in Japanese)
- Fujino, A., Ueda, N., and Saito, K. (2008). Semisupervised
learning for a hybrid generative/discriminative classifier based on the maximum
entropy principle. IEEE Transactions on Pattern Analysis and Machine
Intelligence (TPAMI), 30(3), 424-437. [IEEE
Xplore] [DOI link]
[IEEE Copyright Notice]
- Ueda N, Yamada T and Kuwata S, "Co-clustering Discrete Data Based on the Dirichlet Process Mixture Model", Transaction of Information Processing Society of Japan, Vol.1 No.1 (pp. 60-73), (2008), [Transaction of Information Processing Society of Japan],(in Japanese)
- Iwata, T.,Yamada, T.,and Ueda, N.,:"Collaborative filtering efficiently using purchase orders" Transaction of Information Processing Society of Japan, Vo.49, No.SIG4
(TOM20), pp. 125-134, 2008, (in Japanese).
- Naud A, Usui S, Ueda N and Taniguchi T, "Visualization of documents and concepts in Neuroinformatics with the 3D-SE Viewer", Neuroinformatics, 2007.
- Kuwata S, Ueda N, "One-shot Collaborative Filtering" Transaction
of Information Processing Society of Japan, Vol.48, No.SIG_15(TOM_18),
pp. 153-162, 2007, [Transaction of Information Processing Society of Japan], (in Japanese)
- Kawamae, N., Yamada, T. and Ueda, N.,"Personalized Ranking by Identifying, RelativeInnovators", FIT2007 Letters, Vol.6, pp.99-102, 2007.
- Kuwata, S., and Ueda, N.,"An efficient collaborative filtering algorithm
based on marginal rating distributions," International Journal of
IT & IC, IEEE CIS, Vol.2, No.1, 2007.
- Fujino A., Ueda, N and Saito K, "Semi-supervised Learning of Multi-class Classifiers for Multi-component Data" Transaction of Information Processing Society of Japan, Vol.48, No.SIG_15(TOM_18), pp. 163-175, 2007, [Transactions of IPSJ.], (in Japanese)
- Fujino, A., Ueda, N., and Saito, K., "A hybrid generative/discriminative approach to text classification with additional information", Information Processing & Management, Elisevier, Vol.43, pp.
379-392, 2007.
- Usui,S.,Plames,P.,Nagata,K.,Taniguchi,T.,and Ueda,N., "Keyword extraction,
ranking, and organization for the neuroinfomatics platform," Biosystems,
Elsevier Science, Vol.88, Issue 3, pp. 334-342, 2007, [Biosystems].
- Iwata,T., Saito,K., Ueda,N, Stromsten,S,Griffiths,T., and Tenenbaum J., "Parametric Embedding for Class Visualization", Neural Computation Vol. 19, No. 9, pp. 2536-2556: 2536-2556, 2007.
- Kawamae, N., Yamada, T. and Ueda, N.,"Personalized Ranking by Identifying, RelativeInnovators", FIT2007 Letters, Vol.6, pp.99-102, 2007.
- Ueda, N., and Yamada, T., "Nonparametric Bayes," Journal of Japanese
Applied Mathematics Vol.17, No.3, pp.196-214, 2007.
- Fujino A.,Ueda,N and Saito,K. "Text Classification by Effectively
Using Additional Information Based on Maximum Entropy Principle(Information
Retrieval)" Transaction of Information Processing Society of Japan,
Vol.47, No.10, pp. 2929-2937, 2006, [ Transactions of IPSJ.], (in Japanese).
- Fujino, A., Ueda, N., and Saito, K."A hybrid generative/discriminative classifier design for semi-supervised learning", Journal of JSAICVol.21, No.3, pp.301-309, 2006, (in Japanese).
- Kimura, M., Saito, K., and Ueda, N., "Modeling network growth with
directional attachment and communities," Systems and Computers in
Japan, Vol. 35, No. 8, pp. 1-11, 2004, [Systems and Computers in Japan].
-
Ueda, N. and Saito, K., "Parametric mixture models for multi-topic text," Systems and Computers in Japan, Vol.37, No.2, pp. 56-66, 2006, [Systems and Computers in Japan]
- Ueda, N., "Ensemble Learning," Transactions of IPSJ, CVIM-1036,
(invited) Vol.46, No.SIG15(CVIM 12), pp. 11-20, 2005, [Transaction of Information Processing Society of Japan], (in Japanese)
- Iwata,T., Saito,K., Ueda,N., Stromsten,S,Griffiths,T., and Tenenbaum J.,
"Parametric Embedding for Class Visualization, " Neural Computation, vol.46,no.9, pp. 2337-2346, 2005, (in Japanese).
- Fujino, A., Ueda, N., and Saito, K.., "Semi-supervised learning on hybrid generative/discriminative models," FIT2005 Letters, 2005, (in Japanese).
- Iwata, T., Saito, K., and Ueda, N., "Visualization via posterior preserving embedding "FIT2004 Letters, Vol. 3, pp. 119-120, 2004, (in Japanese).
- Kaneda, Y., Saito, K., and Ueda, N., "Automatic extraction of correspondences between document taxonomies," FIT2004 Letters, Vol. 3, pp.121-122, 2004, (in Japanese).
- Kaneda, Y., Ueda, N., "A Robust text data clustering method for high-dimensional
data," FIT2004 Letters, Vol. 3, pp. 123-124, 2004, (in Japanese).
- Fujino., A., Ueda, N., and Saito, K.., "Relevance feedback with cross
validation," FIT2004, Vol. 3, pp. 53-54, 2004, (in Japanese).
- Kimura, M., Saito, K., and Ueda, N, "Modeling share dynamics by extracting competition structure", Physica D, Vol.198, pp. 51-73, 2004.
- Watanabe, S., Minami, Y., Nakamura, A., and Ueda, N., "Variational Bayesian Estimation and Clustering for Speech Recognition", IEEE transaction on Speech and Audio Processing, Vol. 12, pp. 365-381,
2004.
- Ueda, N., and Inoue, M., "Extended Tied-Mixture HMMs for Both Labeled
and Unlabeled Time Series Data", Journal of VLSI Signal Processing, pp. 189-197, 2004.
- Kimura, M., Saito, K., and Ueda, N, "Modeling of growing networks with directional attachment and communities", Neural Networks, Vol. 17, No. 7, pp. 975-988, 2004.
- Ueda, N, and Saito, K. " Parametric Mixture Models for Multi-Topic
Text", Transactions of IEICEJ, (D-II), Vol. J87-DII, No.3, pp. 872-883,
2004, (in Japanese)
- Ueda, N. and Inoue, M., "Extended tied-mixture HMMs for both labeled
and unlabeled time series data," to appear Journal of VLSI Signal
Processing Systems,Vol. 37, pp. 189-197, 2004.
- Kimura, M., Saito, K., and Ueda, N, "Modeling of growing networks with directional attachment and communities," Transactions of IEICEJ, Vol. J86-DII, No, 10, pp. 1468-1479, 2003, (in Japanese).
- Ueda, N. and Saito, K., "Multi - topic Text Model for Topic - based
Text Retrieval" Transaction of Information Processing Society of Japan
Vol. 44, No. SIG14(TOM9), pp. 1-8, 2003,[Transaction of Information Processing Society of Japan] (in Japanese).
- Yamada, T., Saito, K., and Ueda. N., "Embedding networks data based
on cross-entropy minimization," Transaction of Information Processing
Society of Japan Vol. 44, No. 9, pp. 2401-2408, 2003, [Transaction of Information Processing Society of Japan] (in Japanese).
- Inoue, M. and Ueda, N., "Exploitation of unlabeled sequences in hidden markov models" IEEE Transaction on Pattern Analysis and Machine Intelligence (PAMI), Vol. 25, No. 12, pp1570-1581, 2003.
- Watanabe, S., Minami, Y., Nakamura, A., and Ueda, N., "Selection of
Shared-States Hidden Markov Model Structure Using Bayesian Criterion,"
Transactions of IEICEJ, Vol. J86-DII, No. 6, pp. 776-786, 2003, (in Japanese).
- Ueda, N. and Ghahramani Z., "Bayesian model search for mixture models
based on optimizing variational bounds," Neural Networks, Vol.15,
pp. 1223-1241, 2002.
- Inoue, M. and Ueda, N., "Use of Unlabeled Time Series Data in Hidden Markov Models" Transactions of IEICEJ, Vol. J86-DII, No. 2, pp. 173-183, 2003 (in Japanese).
- Ueda, N., "Variational Bayesian Learning for Optimal Model Search" Journal of Japanese Society for Artificial Intelligence, Vol.16,
No.2, 2001, (in Japanese).
- Suzuki, S., and Ueda, N., "Adaptive clustering method using modular
learning architecture," Transactions of IEICEJ, Vol. J83_DII, No.
6, pp. 1529-1538, 2000, (in Japanese).
- Ueda, N., "EM algorithm with split and merge operations for mixture
models (invited)," Transactions of IEICE, Vol. E83-D, No. 12, pp.
2047-2055, 2000.
- Suzuki, S., and Ueda, N., "Adaptive clustering method using modular learning architecture," Transactions of IEICEJ, Vol. J83_DII, No. 6, pp. 1529-1538, 2000, (in Japanese).
- Ueda, N., Nakano, R., Ghahramani, Z., and Hinton, G. E.,"SMEM Algorithm
for Mixture Models," Neural Computation, Vol. 12, No. 9, pp. 2109-2128,
2000.
- Ueda, N., Nakano, R., Ghahramani, Z., and Hinton, G. E.,"Split and
merge EM algorithm for improving Gaussian mixture density estimates (invited),"
Journal of VLSI Signal Processing, Vol. 26, pp. 133-140, 2000.
- Ueda, N., "Optimal Linear Combination of Neural Networks for Improving Classification Performance," IEEE Transactions on Pattern Analysis and Machine Intelligence (PAMI). Vol. 22, No.2, pp. 207-215, 2000.
- Ueda, N. and Nakano, R., "Probabilistic Mixture Subspace Method,"
Transactions of IEICE, Transactions of IEICEJ, Vol. J82-DII, No. 12, pp.
2394-2401, 1999, (in Japanese).
- Ueda, N. and Nakano, R., "EM Algorithm with Split and Merge Operations
for Mixture Models," Transactions of IEICE, Transactions of IEICEJ,
Vol. J82-DII, No. 5, pp. 930-940, 1999, (in Japanese).
- Ueda, N., "Optimum Linear Combination of Neural Network Classifiers Based on the Minimum Classification Error Criterion," Transactions of IEICEJ, Vol. J82_DII, No. 3, pp. 522-530, 1999, (in Japanese).
- Ueda, N. and Nakano, R., "Deterministic Annealing EM Algorithm,"
Neural Networks, Vol. 11, No. 2, pp. 271-282, (1998).
- Ueda, N. and Nakano, R., "Analysis of Generalization Error on Ensemble Learning," Transactions of IEICEJ, Vol. J80-DII, No. 9, pp. 2512-2521, 1997, (in Japanese).
- Ueda, N. and Nakano, R., "Deteministic Anneling EM Algorithm,"
Transactions of IEICEJ, Vol. J80-DII, No. 1, pp. 267-276, 1997, (in Japanese).
- Ueda, N. and Mase, K., "Tracking Moving Contours Using Energy-minimizing
Elastic Contour Models," International Journal of Pattern Recognition
and Artificial Intelligence, Vol. 9, No. 3, pp. 465-484, 1995.
- Ueda, N. and Nakano, R., "A New Competitive Learning Approach Based on an Equidistortion Principle for Designing Optimal Vector Quantizers,"Neural Networks, Vol.7, No.8, pp. 1211-1227, 1994.
- Ueda, N. and Nakano, R., "Competitive and Selective Learning method
for Vector Quantizer Design - Equidistortion Principle and Its Algorithm
-,"Transactions of IEICEJ, Vol. J77-DII, No. 11, pp. 2265-2278, 1994,
(in Japanese).
- Ueda, N. and Suzuki, S., "Learning Visual Models from Shape Contours
Using Multiscale Convex/Concave Structure Matching," IEEE Transactions
on Pattern Analysis and Machine Intelligence (PAMI), Vol. 15, No. 4, pp.
337-352, 1993, [ IEEE Transactions on Pattern Analysis and Machine Intelligence (PAMI).
- Suzuki, S., Ueda, N. and Sklansky, J., "Graph-Based Thinning for Binary
Images," International Journal of Pattern Recognition and Artificial
Intelligence, Vol. 7, No. 5 pp. 1009-1030, 1993.
- Ueda, N., Mase K., and Suenaga Y., "A Contour Tracking Method Using Elastic Contour Model and Energy Minimization Approach," Transactions of IEICEJ, Vol. J75-DII, No. 1, pp. 111-120, 1992, (in Japanese).
- Ueda, N. and Suzuki, S., "Automatic Shape Model Acquisition Based
on A Generalization of Convex/Concave Structure," Transactions of
IEICEJ, Vol. J74-DII, No. 2, pp. 220-229, 1991, (in Japanese).
- Ueda, N. and Suzuki, S., "A Deformed Line-Drawing Matching Algorithm
Using Multiscale Convex/Concave Structures," Transactions of IEICEJ,
Vol. J73-DII, No. 7, pp. 992-1000, 1990, (in Japanese).
- Ueda, N., Nagura, M., Kosugi, M., and Mori, K., "Image Enhancemen Method for Law Quality Drawings," Transactions of the Institute of Television Engineers of Japan, Vol. 42, No. 8, pp. 831-836, 1988, (in Japanese).
IEEE Copyright Notice
©2008 IEEE. Personal use of this material is permitted. However, permission
to reprint/republish this material for advertising or promotional purposed
or for creating new collective works for resale or redistribution to servers
or lists, or to reuse any copyrighted component of this work in other works
must be obtained from the IEEE.
These materials are presented to ensure timely dissemination of scholarly and
technical work. Copyright and all rights therein are retained by authors or by
other copyright holders. All persons copying this information are expected to
ashere to the terms and constraints invoked by each author's copyright. In most
cases, these works may not be reposted without the explicit permission of the
copyright holder.
Refereed International Conference Papers
- Shiro Usui, Nilton L. Kamiji, Tatsuki Taniguchi, Naonori Ueda, "RAST:
A Related Abstract Search Tool," International Conference on Neural
Information Processing (ICONIP) 2009.
- Tomoharu Iwata, Takeshi Yamada, Naonori Ueda, "Modeling Social Annotation
Data with Content Relevance using a TopicModel,"Advances in Neural
Information Processing Systems (NIPS2009), 835-843, 2009
- Tomoharu Iwata, Shinji Watanabe, Takeshi Yamada, Naonori Ueda,"Topic
Tracking Model for Analyzing Consumer Purchase Behavior,"Proc. of
21st International Joint Conference on Artificial Intelligence(IJCAI-09),
1427-1432, 2009
- Tomoharu Iwata, Takeshi Yamada, Naonori Ueda, "Probabilistic Latent Semantic Visualization: Topic Model for Visualizing Documents," Proc. of 14th ACM SIGKDD International Conference on Knowledge
Discovery and Data Minig (KDD2008), pp.363-371, 2008.
- Ishiguro, K., Yamada, T. and Ueda, N, "Simultaneous Clustering and Tracking Unknown Number of Objects", Proc. of the 19th IEEE Computer Society Conference on Computer Vision and Pattern Recognition (CVPR08), pp. 1-8, 2008, [CVPR08]
- Usui S, Naud A, Ueda N and Taniguchi T, "3D-SE Viewer: A Text Mining
Tool based on Bipartite Graph Visualization", 20th International Joint
Conference on Neural Networks (IJCNN'07), 2007.
- Kuwata S. and Ueda N., "One-shot Collaborative Filtering," IEEE CIDM2007, Vol.1, No.1, pp.300-307, 2007.
- Fujino A., Ueda N., and Saito K., "Semi-supervized learning for multi-component
data classification," to appear in Proc. of International Joint Conference
on Areificial Intelligence (IJCAI2007),2007.
- Kuwata S. and Ueda N., "One-shot collaborative filtering," to
appear in Pro. of IEEE Symposium on Compututational Intelligence and Data
Mining (CIDM2007), 2007.
- Kemp C., Tenenbaum J. B., Griffiths T.L.., Yamada T. and Ueda N., "Learning systems of concepts with an infinite relational model," Proc. of the 21st National Conference on Artificial Intelligence(AAAI-06), 2006
- Iwata T., Saito K., and Ueda N., "Visual nonlinear discriminant analysis for classifier design," Proc. of the 14th European Symposium on Artificial Neural Networks
(ESANN2006),pp.283-288, 2006.
- Usui S., Palmes P., Nagata K., Taniguchi T. and Ueda N, "Extracting
keywords from research abstracts for the neuroinformatics platform index
tree," To appear in Proc. of International Joint Conference on Neural
Networks (IJCNN2006),
2006.
- Ueda N., "Bayesian probabilistic models for data partitioning and
their applications," Proc. of the 17th International Symposium on
Mathematical Theory of Networks and Systems (MTNS2006), 2006.
- Saito K.. and Ueda N., "Filtering Search Engine Spam based on Anomaly
Detection Approach," Proc. of the KDD2005 Workshop on Data Mining
Methods for Anomaly Detection, pp.62-67, 2005.
- Usui S., Palmes P., Nagata K., Taniguchi T. and Ueda N., "Relevance
keyword extraction, ranking, and organization for the neuroinformatics
platform, Proc. of International Conference on Biological Computation,"
Proc. of BIOCOMP, 2005.
- Fujino A.., Ueda N., and Saito K., "A Classifier design based on combining
multiple components by maximum entropy principle," Proc. of the 2nd
Asia Information Retrieval Symposium (AIRS2005), 2005.
- Kimura M., Saito K., and Ueda N., "Multinomial PCA for extracting
major latent topics from document streams," Proc. of IJCNN, 2005.
- Fujino A.., Ueda N. and Saito K.., "A hybrid generative/discriminative
approach to semi-supervised classifier design," Proc. of the 20th
National Conference on Artificial Intelligence (AAAI-05), pp. 764-769,
2005.
- Inoue M. and Ueda N., "Retrieving lightly annotated images using image
similarities," ACM Symposium on Applied Computing (SAC), Special Track
on Information Access and Retrieval (IAR)Santa Fe, March 14-17, pp.1031-1037,
2005
- Iwata T, Saito K, Ueda N, Stromsten S, Thomas L. Griffiths, Joshua B. Tenenbaum, "Parametric Embedding for Class Visualization, " Advances in Neural Information Processing Systems 17 (NIPS2004), pp. 617-624, 2005
- Kimura M., Saito K. and Ueda N., "Modeling share dynamics by extracting competition structure," Proc. of the 5th International Conference on Complex Systems, p. 72, 2004.
- Kaneda Y., Ueda N. and Saito, K., "Extended Parametric Mixture Model
for Robust Multi-labeled Text Categorization," Proc. of the 8-th International
Conference on Knowledge-Based Intelligent Information & Engineering
Systems, Vol. 3214 of Lecture Notes in Computer Science, pp. 616-623, 2004.
- Ueda N., and Saito K., "Simplex mixture models for multi-topic text," In Science of Modeling, ISM Report on Research and Education No.17, The Institute of Statisitical Mathematics, pp. 380-381, 2003.
- Kimura M., Saito K. and Ueda N., "Modeling share dynamics by extracting competition structure," In Science of Modeling, ISM Report on Research and Education No.17, The Institute of Statisitical Mathematics, pp.366-367, 2003.
- Yamada T., Saito K and Ueda N, "Cross-entropy based embedding for relational data," International Conference on Machine Learning (ICML2003), pp. 832-839, 2003.
- Watanabe S., Minami Y., Nakamura A., and Ueda N., "Bayesian acoustic modeling for spontaneous speech recognition," IEEE Workshop on Spontaneous Speech Processing and Recognition (SSPR03), pp. 47-50, 2003.
- Watanabe S., Minami Y., Nakamura A., and Ueda N., "Application of variational bayesian estimation and clustering to acoustic model adaptation," IEEE International Conference on Acoustic, Speech, and Signal Processing (ICASSP03), Vol. 1, pp. 568-571, 2003.
- Kimura M., Saito K., and Ueda N., "Modeling of growing networks with directional attachment and communities," European Symposium on Artificial Neural Networks (ESANN03), pp. 15-20, 2003.
- Kimura M., Saito K., and Ueda N., "Modeling of
growing networks with communities," IEEE International Workshop on Neural
Networks for Signal Processing (NNSP2002),
pp. 189-198, 2002.
- Watanabe S., Minami Y., Nakamura A., and Ueda N., "An application of variational Bayesian approach to speech recognition," to appear Advances in Neural Information Processing Systems 15(NIPS15), MIT Press, pp. 1261-1268, 2002.
- Watanabe S., Minami Y., Nakamura A., and Ueda N., "Constructing shared-state
HMMs based on a Bayesian approach," International Conference on Spoken
Language Processing (ICSLP02), Vol. 4, pp. 2669-2672, 2002.
- Ueda N. and Saito K., "Parametric mixture models for multi-topic text," Neural Information Processing Systems 15(NIPS15), MIT Press, pp. 737-744, 2002.
- Ueda N. and Saito K., "Singleshot detection of multi-category text using parametric mixture models," ACM SIG Knowledge Discovery and Data Mining (SIGKDD2002), pp. 626-631, 2002.
- Inoue M. and Ueda N., " HMMs for both labeled and unlabed time series
data," IEEE Neural Networks for Signal Processing (NNSP2001), pp.
93-102, 2001.
- Ueda N. and Ghahramani Z., "Optimal model inference for Baysian mixture
of experts," IEEE Neural Networks for Signal Processing (NNSP2000),
pp. 145-154, 2000.
- Ueda N., Nakano R., Ghahramani Z. and Hinton, G. E., "Pattern classification using a mixture of factor analyzers," IEEE Neural Networks for Signal Processing (NNSP99), pp. 525-533, 1999.
- Ueda N., Nakano R., Ghahramani Z., and Hinton G. E., "SMEM algorithm for mixture models," Neural Information Processing Systems 11 (NIPS11),
pp. 599-605, 1999.
- Ueda N., Nakano R., Ghahramani Z. and Hinton G. E., "Split and merge EM algorithm for improving Gaussian mixture density estimates," IEEE Neural Networks for Signal Processing (NNSP98), pp. 274-283, 1998.
- Ueda N. and Nakano R., "Combining discriminant-based classifiers using
the minimum classification error discrimininant,"IEEE Neural Networks
for Signal Processing(NNSP97), pp. 365-374, 1997.
- Suzuki S. and Ueda N., "Self-organization of feature columns and its
application to object classification," Proceedings of International
Conference on Neural Information Processing (ICONIP97), pp. 1166-1169,
1997.
- Ueda N. and Nakano R., "Generalization error of ensemble estimators,"
Proceedings of International Conference on Neural Networks (ICNN96), pp.
90-95, 1996.
- Ueda N. and Nakano R., "Deterministic annealing variant of the EM algorithm," Neural Information Processing Systems 7 (NIPS7), MIT Press,
Cambridge MA, pp. 545-552, 1995.
- Ueda N. and Nakano R., "A new maximum likelihood training and application to probabilistic neural networks," Proceedings of International Conference on Artificial Neural Networks (ICANN95), pp. 497-504, 1995.
- Ueda N. and Nakano R., "Estimating expected error rates of neural
network classifiers in small sample size situations: A comparison of cross-validation
and bootstrap," International Conference on Neural Networks (ICNN95),
pp. 101-104, 1995.
- Ueda N. and Nakano R., "Mixture density estimation via EM algorithm
with deterministic annealing," Proceedings of IEEE Neural Networks
for Signal Processing (NNSP94)}, pp. 69-77, 1994.
- Ueda N. and Nakano R., "A new learning approach based on equidistortion principle for optimal vector quantizer Design," Proceedings of IEEE Neural Networks for Signal Processing (NNSP93), pp. 362-371, 1993.
- Ueda N. and Nakano R., "Competitive and selective learning method
for designing optimal vector quantizers," Proceedings of IEEE International
Conference on Neural Networks (ICNN93), pp. 1444-1450, 1993.
- Ueda N. and Mase K., "Tracking moving contours using energy-minimizing
elastic contour models,"Proceedings of European Conference on Computer
Vision (ECCV92), pp. 453-457, 1992.
- Suzuki S. and Ueda N., "Robust vectorization using graph-based thnning and reliability-based line approximation," Proceedings of IEEE Conference on Computer Vision (CVPR91), pp. 494-500, 1991.
- Ueda N. and Suzuki S., "Automatic shape model acquisition using multiscale
segment matching," Proceedings of International Conference on Pattern
Recognition (ICPR90), pp. 897-902, 1990.
- Ogawa H., Kawada E.. and Ueda N., "Application of image processing
equipment with multiprocessors to line-drawing recognition," Proceedings
of SPIE-845, pp. 97-103, 1987.
- Okudaira M., Ueda N. and Aoki U., "Image enhancement of handwritten
drawings and their recognition followed by interactive processing,"
Proceedings of SPIE-707, pp. 42-50, 1986.
Invited Paper
- Ueda, N., Nakano, R., "Competitive and selective learning method for vector quantizer design -Equidistortion principle and
its algorithm," Systems and computers in Japan, Scripta Technica,
Inc., Vol. 26, No. 9, pp. 34-49, 1995.
- Ueda, N., Mase, K. and Suenaga, Y., "A contour tracking method using
elastic contour model and energy minimizing approach," Systems
and computers in Japan, Scripta Technica, Inc., Vol. 24, No. 8, pp. 59-70, 1993.
- Ueda, N. and Suzuki, S., "Automatic shape model acquisition based on a generalization of convex/concave
structure," Systems and computers in Japan, Scripta Technica, Inc. Vol. 23, No. 1, pp. 89-100, 1992.
- Ueda, N. and Suzuki, S., "A matching algorithm of deformed planar curves using multiscale convex/concave
structures," Systems and computers in Japan, Scripta Technica,
Inc. Vol. 22, No. 5, pp. 94-104, 1991.
Invited Tutorial Papers
- Ueda, N., "EM algorithm," Society of Instrument and Control Engineers(SICE), Vol.44, No.5, pp.333-338, 2005. (In Japanese)
- Ueda, N., "The goal of Web science research," OHM, Vol.91, No.10,pp.6--7, 2004.(In Japanese)
- Ueda, N., "Bayesian Inference Algorithms -Approximation Methods for High-Dimensional Integrals-," Journal of the Japanese Society for Artificial Intelligence, Vol. 19, No. 6, 2004 (in Japanese).
- Ueda, N. and Saito, K., "Probablistic Models for Multi-topic Text," Information Processing Society of Japan,
Vol. 45, No. 2, 3, 2004 (in Japanese).
- Ueda, N., "Probablistic Models and Statistical Learning," Computer Today, No.114, 2003.
- Ueda, N., "Bayeaian Learning I - IV," Journal of IEICEJ, Vol. 85, No. 4, 6, 7, 8, 2002 (in Japanse).
- Ueda, N., "Ensemble Learning," Journal of the Society of Instrument and Control Engineers, Vol. 41, pp. 248, 2002.
- Ueda, N., "The Front of Bayesian Learning -Variational Bayesian Learning,
" Information Processing Society of Japan, Vol. 42, No. 1, 2001 (in Japanese).
- Ueda, N., "An Inquiry into Statistical Learning Research," Information
Processing Society of Japan,, Vol. 41, No. 6, pp. 730-733, 2000 (in Japanese).
- Ueda, N. and Nakano, R., "Deterministic Annealing EM Algorithm," Journal of
the Society of Instrument and Control Engineers, Vol. 38, No. 7, pp. 444-449, 1999 (in Japanse).
- Ueda, N. and Nakano, R., "Deterministic Annealing -Another Type of Annealing-," Journal of Japanese Society for Artificial Intelligence, Vol. 12, No. 5, pp. 689-697, 1997 (in Japanse).
- Mase, S. and Ueda, N., "Mathematical Morphology and Image Analysis I," Journal of IEICEJ,
Vol. 64, No. 2, pp. 166-174 , 1991 (in Japanese).
- Ueda, N. and Mase, S., "Mathematical Morphology and Image Analysis
II," Journal of IEICEJ, Vol. 64, No. 3, pp. 271-279, 1991 (in Japanse).
Internal Invited Technical Reports at NTT
- Fujino, A., Ueda, N., and Saito, K., "Semi-supervised learning forautomatic text classification,
" NTT Technical Journal, Vol.19, No.6, pp.26-28, 2007. (In Japanese)
- Ueda, N., "Web Science Research," NTT Technical Review}, Vol.
3, No. 3, pp. 12-14, 2005.
- Ueda, N., "Comtemplation to Web science," NTT Technical Journal, Vol.16,No.6, p. 22, 2004. (In Japanese)
- Ueda, N., "Web Science Research," NTT Technical Journal, Vol.16, No.6,2004. (In Japanese)
- Ueda, N. and Nakano, R., "Competitive and Selective Learning Method for Optimal Vector Quantizer Design," NTT R&D, Vol. 42, No. 6, 1993 (in Japanese).
- Nakano, R., Ueda, N., Saito, K., and Yamada, T., " Research on Learning Aiming at Artificial Intelligence," NTT R&D, Vol. 42, No. 9, pp. 1175-1184, 1993 (in Japanese).
- Ueda, N., Mase, K., and Suenaga, Y., "Contour Tracking Method Using Energy-minimizing Elastic Models,"
NTT R&D, Vol. 42, No. 4, pp. 477-486, 1993 (in Japanese).
- Ueda, N. and Suzuki, S., "Multiscale Convex/Concave Structure Matching : MC Matching Method,"
NTT R&D, Vol. 40, No. 3, pp.399-406, 1991 (in Japanese).
- Kawada, E, Ueda, N., Ogawa, H., and Kosugi, M., "A Figure Recognition
Method for Hand-Written Drawings," NTT Electrical Communications Laboratories
Technical Journal, Vol. 37, No. 3, pp. 217-223, 1988 (in Japanese).
- Ueda, N., Nagura, M., Hoshino, T., and Mori, K., "Image Enhancement Method for Hand-Written Line Drawings,"
NTT Electrical Communications Laboratories Technical Journal, Vol. 37,
No. 3, pp. 211-216, 1988 (in Japanese).
Book & Book Chapters
- Kabashima, Y. and Ueda, N., "Frontier of Statistical Science 11, Computational
Statistics I - (3)," Iwanami Shoten, Japan, 2003.(in Japanese)
- Ishii, K., Ueda, N., Maeda, E., and Murase, H., "Introduction to Pattern
Recognition," Ohm-Sha, Tokyo, Japan, 1998.(in Japanese)
- Ueda, N., "Chapter 3: Pattern Recognition Theory," in IEICEJ
(Ed.),"Handbook of Electronics, Information and Communication,"
Asakura-Shoten, Tokyo, Japan, 1998.(in Japanese)
- Ueda, N., "Chapter 9: Vector Quantization," in Amari, S. (Ed.),"Handbook
of Brain Science," Asakura-Shoten, Tokyo, Japan, 1995.(in Japanese)
- NTT Human Interface Laboratory, Project RTV, Japanese translation of "Horn,
B. K. P., Robot Vision, MIT-Press, 1986" Asakura-Shoten, Tokyo, Japan,
1993. (in Japanese)