|
 |
Listening to human speech in noisy reverberant environments |
 |
Audio communication scene analysis by computer |
  |
Speech is one of the most natural and useful media for human communication. If a computer could appropriately handle speech signals in our daily lives, it could provide us with more convenient and comfortable speech services. However, when a speech signal is captured by distant microphones, background noise and reverberation contaminate the original signal and severely degrade the performance of existing speech applications. To overcome such limitations, we are investigating methodologies for automatically detecting individual speech signals in captured signals (scene analysis) and recovering their original quality (speech enhancement). Our research goal is thus to establish techniques that extract such information as "who spoke when" from human communication scenes and enable various speech applications to work appropriately in the real world.
|
  |
* Future applications
For existing speech applications, close-talk microphones are essential for reducing the influence of background noise and reverberation. Obviously, using such microphones is not convenient in our daily lives. In contrast, if a computer can automatically analyze communication scenes using distant microphones and precisely extract individual speech signals, we can eliminate the need for close-talk microphones for such applications. For example, we will be able to control electrical appliances from a distance by speaking, and talk to autonomous robots. For an automatic meeting-minutes generation system, we may not need microphones for individual speakers, but only a microphone array in the meeting room. For human-human communications, the influence of noise and reverberation must be reduced to improve speech intelligibility. Such techniques can be applied to remote conference and mobile communication systems.
|
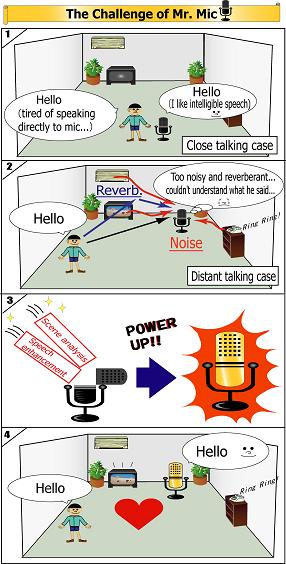 |
  |
* Speaker indexing in a meeting
Techniques for understanding audio scenes are needed to allow us to establish more convenient speech communication. We are developing a method for speaker indexing that estimates "who spoke when" in a meeting with multiple participants. This technique is realized with a noise robust voice activity detection (VAD) technique that estimates "when the utterance was spoken" and a direction of arrival (DOA) estimation technique that estimates "from which direction the utterance was spoken".
|
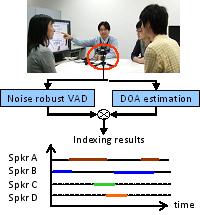 |
  |
* Statistical model based speech enhancement
Speech enhancement, including noise reduction and dereverberation, is a key technology for achieving accurate automatic speech recognition (ASR) in a real world environment. Although many enhancement methods have already been proposed, most of them could not efficiently improve the ASR performance, since there is often a mismatch between speech processed using these methods and the speech model in the ASR system. To solve this problem and obtain appropriate results, we investigate enhancement algorithms that guide their outputs according to the statistical speech model.
|
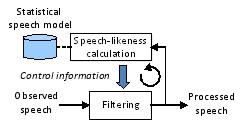 |
  |
* Unified framework of audio signal processing
What we hear in our daily lives consists of a variety of sounds from different sources. This research is aimed at decomposing such complicated sound mixtures into a set of individual source factors and propagation factors, which characterize the direction and reverberation of each source. This technology serves as a tool for unifying different types of audio signal processing such as acoustic noise reduction, dereverberation, and sound source localization. The outcome of this research has been used in our meeting analysis system.
|
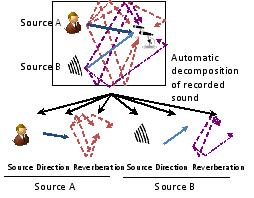 |
  |
[ Reference ] |
[1] T. Yoshioka, T. Nakatani, M. Miyoshi, and H. G. Okuno,
"Blind separation and dereverberation of speech mixtures by joint
optimization," IEEE Transactions on Audio, Speech, and Language Processing,
vol. 19, no. 1, pp. 69-84, Jan. 2011.
|
[2] K. Kinoshita, M. Souden, M. Delcroix and T. Nakatani,
"Single channel dereverberation using example-based speech enhancement with
uncertainty decoding technique,'' Proc. of Interspeech2011 , pp.197-200, 2011.
|
[3] T. Nakatani, S. Araki, T. Yoshioka, M. Delcroix, and M. Fujimoto,
"Dominance Based Integration of Spatial and Spectral Features for Speech
Enhancement," IEEE Trans. ASLP., vol. 21, No. 12, pp.2516-2531, Dec. 2013.
|
[4] M. Souden, K. Kinoshita, M. Delcroix, and T. Nakatani,
"Location Feature Integration for Clustering-Based Speech Separation in
Distributed Microphone Arrays," IEEE Trans. ASLP, Vol. 22, no. 2, pp.
354-367, 2014.
|
[5] N. Ito, S. Araki, and T. Nakatani, "Probabilistic Integration of Diffuse
Noise Suppression and Dereverberation, " Proc. ICASSP, 2014.
|
[6] M. Fujimoto, Y. Kubo, and T. Nakatani, "Unsupervised non-parametric
Bayesian modeling of non-stationary noise for model-based noise
suppression," in Proc. ICASSP, 2014.
|
[7] A. Ogawa, K. Kinoshita, T. Hori, T. Nakatani and A. Nakamura,
"Fast segment search for corpus-based speech enhancement based on speech
recognition technology," in Proc. ICASSP, 2014.
|
[8] K. Kinoshita, M. Delcroix, T. Yoshioka, T. Nakatani, E. Habets, R.
Haeb-Umbach, V. Leutnant, A. Sehr, W. Kellermann, R. Maas, S. Gannot, B.
Raj, B, "The REVERB challenge: a common evaluation
framework for dereverberation and recognition of reverberant speech,"
in Proc. WASPAA, 2013.
|
[9] T. Yoshioka, A. Sehr, M. Delcroix, K. Kinoshita, R. Maas, T. Nakatani,
and W. Kellermann, "Making machines understand us in reverberant rooms:
robustness against reverberation for automatic speech recognition," IEEE
Signal Processing Magazine, vol. 29, no. 6, pp. 114-126, Nov. 2012.
|
|