Big Data Analysis
Understanding stream data from essential elements
- Feature selection for high-dimensional time series data -
Abstract
In this research, we develop a pattern mining technique from high-dimensional time series data. Given the time series data, our technique finds hidden segments of time steps called “states” or “patterns”. Furthermore, our technique automatically extracts “essential” features that characterize the patterns. The technique is especially suitable for the analysis of time series data containing many noisy features because it automatically excludes these noisy features, which are not responsible for pattern changes. The technique will also reduce the number of man-hours needed to analyze the time series data because it selects the essential features of time series patterns to interpret the analysis results.
Poster
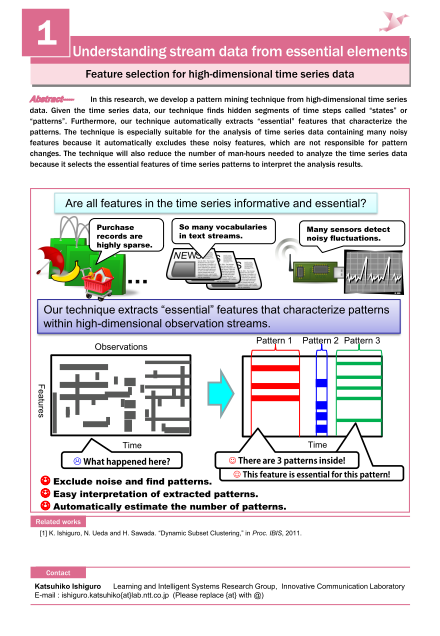
Please click the thumbnail image to open the full-size PDF file.
Presentor