Probabilistic latent variable models have successfully captured the intrinsic characteristics of various data. Understanding them is helpful for discovering latent rules and facts behind data. However, it is nontrivial to design appropriate models for given data because both machine learning and domain-speci?c knowledge are required.
We propose an automatic model generation method for data with hierarchical structure. Our method constructs an appropriate model for given data by extracting important hierarchies and preserves hierarchical and sequential information if needed or desired. We automatically extract latent structures of given data and discover hidden rules and facts behind the data.
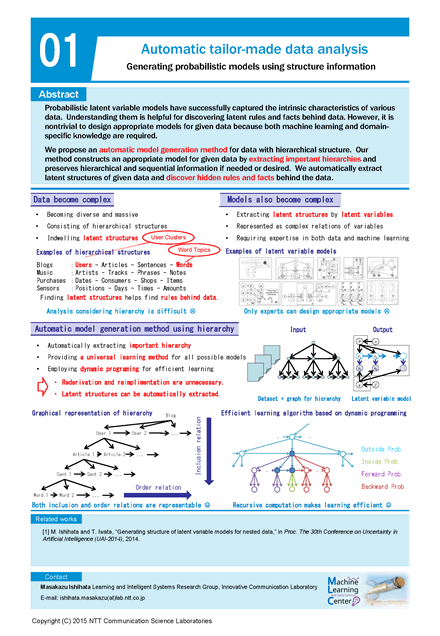
Please click the thumbnail image to open the full-size PDF file.