Papers
- Hirokazu Kameoka, Li Li, Shota Inoue, Shoji Makino, "Semi-blind source separation with multichannel variational autoencoder," arXiv:1808.00892 [stat.ML], Aug. 2018.
Multichannel variational autoencoder (MVAE)
MVAE is a multichannel source separation method that uses a conditional variational autoencoder (CVAE) to model and estimate the power spectrograms of the sources in a mixture. By training the CVAE using the spectrograms of training examples with source-class labels, we can use the trained decoder distribution as a universal generative model that is able to generate spectrograms conditioned on a specified class label. By treating the latent space variables and the class label as the unknown parameters of this generative model, we can develop a convergence-guaranteed semi-blind source separation algorithm that consists of iteratively estimating the power spectrograms of the underlying sources as well as the separation matrices.
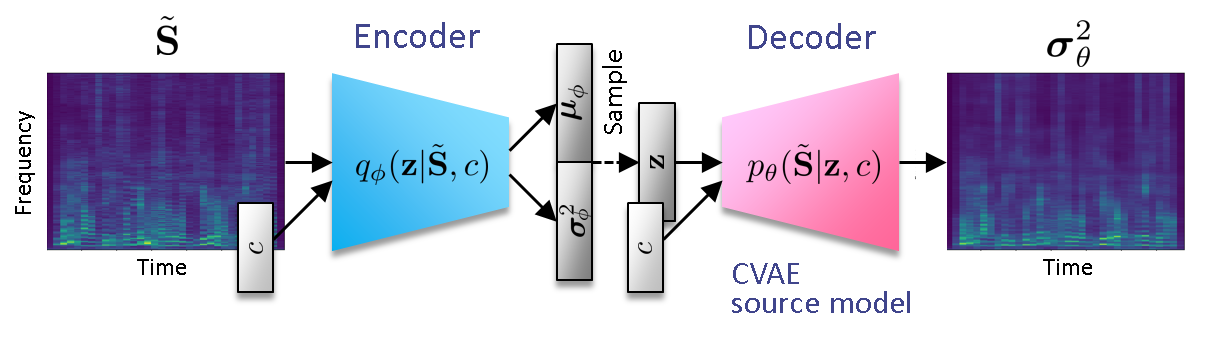
Audio examples
Here, we demonstrate audio examples of MVAE tested on a multichannel source separation task. We selected speech of two female speakers, 'SF1' and 'SF2', and two male speakers, 'SM1' and 'SM2', from the Voice Conversion Challenge (VCC) 2018 dataset [3] for training and evaluation.
SF1 (Female) + SF1 (Female) pair
Mixture signal 1 (Channel 1) | Mixture signal 2 (Channel 2) |
---|---|
Separated signal 1 (MVAE) | Separated signal 2 (MVAE) |
---|---|