Demonstrations of Underdetermined Blind Separation of Speech Signals
last modified: Jan. 13, 2004.
Contents
1: Abstract
2: References
3: Results (simulated anechoic mixtures)
4: Results (echoic mixtures)
5: Discussions
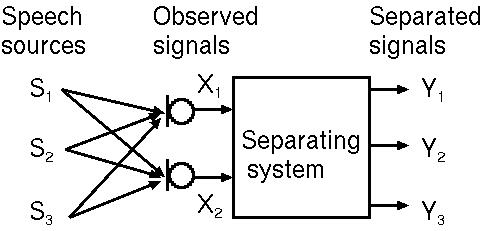
Fig. 1: Block diagram of underdetermined BSS. |
We propose a method for separating speech signals with little distortion
when the signals outnumber the sensors.
Several methods have already been proposed
for solving the underdetermined problem,
and some of these utilize the sparseness of speech signals.
These methods employ binary masks that extract a signal at time points
where the number of active sources is estimated to be only one.
However, these methods result in an unexpected excess of zero-padding and
so the extracted speeches are severely distorted and have loud musical noise.
Moreover, the performance depends on the heuristic parameter of mask width.
To overcome this problem,
we have proposed combining a binary mask and
independent component analysis (ICA).
We call this method BMICA.
First, using sparseness,
we estimate the time points when only one source is active.
Then,
we remove this single source with a wide binary mask from the observations
and apply ICA to the remaining mixtures.
Because the single source removal of this method cause less
discontinuous zero-padding
than in the binary masks only method, we have been able to obtain
separated signals with little distortion with our method.
However,
as our method has still employed a binary mask for one source removal,
the zero-padding to the separated signals has still remained.
Moreover,
there are heuristic parameters to design the shapes of binary masks
in the conventional methods.
Here, we propose a new method
which employs a directivity pattern based continuous mask (DCmask) in the 1st stage.
The DCmask has a small gain for the DOA of one source and preserves signals from other directions.
First we estimate the DOA of each sources with sparseness.
Then, we remove single source from the observations with a DCmask.
Then apply ICA to the remaining mixtures as our former method.
Because this DCmask has no zero in their frequency characteristic,
discontinuous zero-padding of the extracted signals does not occur by nature.
Moreover, we do not need any parameters for designing the DCmask.
Experimental results show that our proposed method can
separate signals with little distortion even in a reverberant condition
without any serious deterioration in the separation performance SIR.
back
- About this method
- S. Araki, S. Makino, H. Sawada and R. Mukai,
``Underdetermined Blind Speech Separation with Directivity Pattern based Continuous Mask and ICA,'' EUSIPCO2004, pp.1991--1994, Sept. 2004.[pdf]
- About the Former Method (BMICA)
-
S. Araki, S. Makino, A. Blin, R. Mukai and H. Sawada,``Blind Separation of More Speech than Sensors with Less Distortion by Combining Sparseness and ICA,'' IWAENC2003, pp.271--274, 2003. [pdf] -->demo page
- About the Sparseness Assessments
- A. Blin, S. Araki and S. Makino,``Blind Source Separation when Speech Signals Outnumber Sensors using a Sparseness-Mixing Matrix Estimation,'', IWAENC2003, pp. 211-214, 2003. [pdf]
back
We simulated an omni-directional microphone pair of
an inter-element spacing of 4 cm giving some delay to the original speech
signals.
The values of delay corresponded to the speech signals from three directions,
45 deg.(s1), 90 deg.(s2), and 135 deg. (s3).
The sampling rate was 8 kHz.
The original speech signals were selected
from the ASJ continuous speech corpus.(All speech signals are in Japanese....Sorry...)
In the tables,
- Sparse: with only binary masks,
- BMICA23: with BMICA (s1 was removed in the 1st stage and s2 and s3 were separated in the 2nd stage),
- BMICA12: with BMICA (s3 was removed in the 1st stage and s1 and s2 were separated in the 2nd stage).
- DCICA23: with NEW method (s1 was removed in the 1st stage and s2 and s3 were separated in the 2nd stage),
- DCICA12: with NEW method(s3 was removed in the 1st stage and s1 and s2 were separated in the 2nd stage).
In the tables,
the values show the separation performance, (SIR, SDR) in dB.
SIR: Signal to Interference Ratio, SDR: Signal to Distortion Ratio.
- female-female-female combination
- original speech:
s1,
s2,
s3
- mixtures:
mic1,
mic2
- separated signals:
|
y1 |
y2 |
y3 |
Sparse |
(21.3, 9.3)
y1 |
(9.7, 13.9)
y2 |
(20.5, 9.6)
y3 |
BMICA23 |
- |
(6.8, 16.3)
y2 |
(13.2, 21.3)
y3 |
BMICA12 |
(13.6, 18.9)
y1 |
(6.8, 16.6)
y2 |
- |
DCICA23 |
- |
(5.4, 14.1)
y2 |
(16.8, 17.2)
y3 |
DCICA12 |
(17.3, 16.0)
y1 |
(5.9, 13.1)
y2 |
- |
- male-male-male combination
- original speech:
s1,
s2,
s3
- mixtures:
mic1,
mic2
- separated signals:
|
y1 |
y2 |
y3 |
Sparse |
(14.4, 5.5)
y1 |
(5.5, 9.2)
y2 |
(17.4, 6.0)
y3 |
BMICA23 |
- |
(3.7, 12.4)
y2 |
(11.3, 12.4)
y3 |
BMICA12 |
(9.2, 16.8)
y1 |
(2.5, 14.7)
y2 |
- |
DCICA23 |
- |
(2.6, 11.5)
y2 |
(14.6, 11.9)
y3 |
DCICA12 |
(12.6, 13.5)
y1 |
(1.4, 11.7)
y2 |
- |
- female-male-male combination
- original speech:
s1,
s2,
s3
- mixtures:
mic1,
mic2
- separated signals:
|
y1 |
y2 |
y3 |
Sparse |
(18.4, 9.0)
y1 |
(11.6, 11.5)
y2 |
(17.3, 9.4)
y3 |
BMICA23 |
- |
(7.9, 13.9)
y2 |
(14.6, 18.5)
y3 |
BMICA12 |
(15.0, 18.7)
y1 |
(8.4, 14.4)
y2 |
- |
DCICA23 |
- |
(5.7, 12.8)
y2 |
(17.3, 17.2)
y3 |
DCICA12 |
(18.5, 16.0)
y1 |
(7.2, 12.6)
y2 |
- |
back
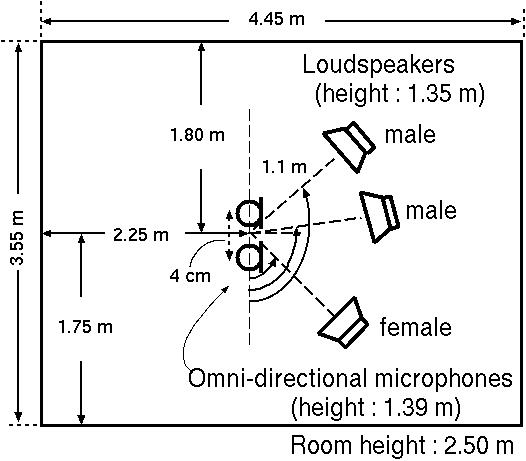
Fig. 2: Room for echoic tests. |
For the echoic tests,
we used speech data
convolved with impulse responses recorded in a real room (wee Fig.2)
whose reverberation time was 130ms.
TR=130ms
- female-female-female combination
- original speech:
s1,
s2,
s3
- mixtures:
mic1,
mic2
- separated signals:
|
y1 |
y2 |
y3 |
Sparse |
(16.0, 6.4)
y1 |
(9.2, 16.0)
y2 |
(13.7, 6.9)
y3 |
BMICA23 |
- |
(7.9, 16.5)
y2 |
(9.7, 10.6)
y3 |
BMICA12 |
(12.2, 8.6)
y1 |
(7.9, 17.6)
y2 |
- |
DCICA23 |
- |
(5.7, 13.1)
y2 |
(13.6, 9.5)
y3 |
DCICA12 |
(15.1, 7.3)
y1 |
(4.8, 7.2)
y2 |
- |
- male-male-male combination
- original speech:
s1,
s2,
s3
- mixtures:
mic1,
mic2
- separated signals:
|
y1 |
y2 |
y3 |
Sparse |
(10.6, 3.7)
y1 |
(2.9, 12.5)
y2 |
(8.6, 4.4)
y3 |
BMICA23 |
- |
(2.2, 12.7)
y2 |
(8.9, 7.9)
y3 |
BMICA12 |
(7.7, 5.9)
y1 |
(2.5, 14.3)
y2 |
- |
DCICA23 |
- |
(1.6, 5.1)
y2 |
(8.2, 5.6)
y3 |
DCICA12 |
(10.9, 6.8)
y1 |
(1.5, 12.2)
y2 |
- |
- female-male-male combination
- original speech:
s1,
s2,
s3
- mixtures:
mic1,
mic2
- separated signals:
|
y1 |
y2 |
y3 |
Sparse |
(10.4, 4.8)
y1 |
(6.8, 13.3)
y2 |
(10.7, 6.2)
y3 |
BMICA23 |
- |
(6.4 14.4)
y2 |
(8.9, 9.5)
y3 |
BMICA12 |
(9.5, 8.8)
y1 |
(6.1, 15.9)
y2 |
- |
DCICA23 |
- |
(4.8 11.7)
y2 |
(10.6, 8.9)
y3 |
DCICA12 |
(11.0, 7.2)
y1 |
(3.1, 6.5)
y2 |
- |
back
-
With a binary mask only, the SIR values were high but the SDR values
were unsatisfactory.
-
In contrast, with our NEW method,
we were able to obtain high SDR values
without any serious deterioration in the separation performance SIR.
-
Even in a reverberant environment,
we obtained reasonable results with our proposed method.
-
It should be noted that
it is hard to separate the signal at the center position by both methods.
-
Although we consider the case of two sensors and three sources here,
we can expand our DCmask method to the underdetermined case of
N \leq (the number of nulls formed by M sensors)
+ (the number of outputs of a standard ICA) = (M-1) + M,
where N and M are the number of sources and sensors, respectively.
back