Please check out our relevant work!
Follow-up work: Previous work: Other relevant work:- StarGAN-VCs: StarGAN-VC, StarGAN-VC2
- Other VCs: Links to demo pages
CycleGAN-VC3
Non-parallel voice conversion (VC) is a technique for learning mappings between source and target speeches without using a parallel corpus. Recently, CycleGAN-VC [1] and CycleGAN-VC2 [2] have shown promising results regarding this problem and have been widely used as benchmark methods. However, owing to the ambiguity of the effectiveness of CycleGAN-VC/VC2 for mel-spectrogram conversion, they are typically used for mel-cepstrum conversion even when comparative methods employ mel-spectrogram as a conversion target. To address this, we examined the applicability of CycleGAN-VC/VC2 to mel-spectrogram conversion. Through initial experiments, we discovered that their direct applications compromised the time-frequency structure that should be preserved during conversion. To remedy this, we propose CycleGAN-VC3, an improvement of CycleGAN-VC2 that incorporates time-frequency adaptive normalization (TFAN). Using TFAN, we can adjust the scale and bias of the converted features while reflecting the time-frequency structure of the source mel-spectrogram. We evaluated CycleGAN-VC3 on inter-gender and intra-gender non-parallel VC. A subjective evaluation of naturalness and similarity showed that for every VC pair, CycleGAN-VC3 outperforms or is competitive with the two types of CycleGAN-VC2, one of which was applied to mel-cepstrum and the other to mel-spectrogram.
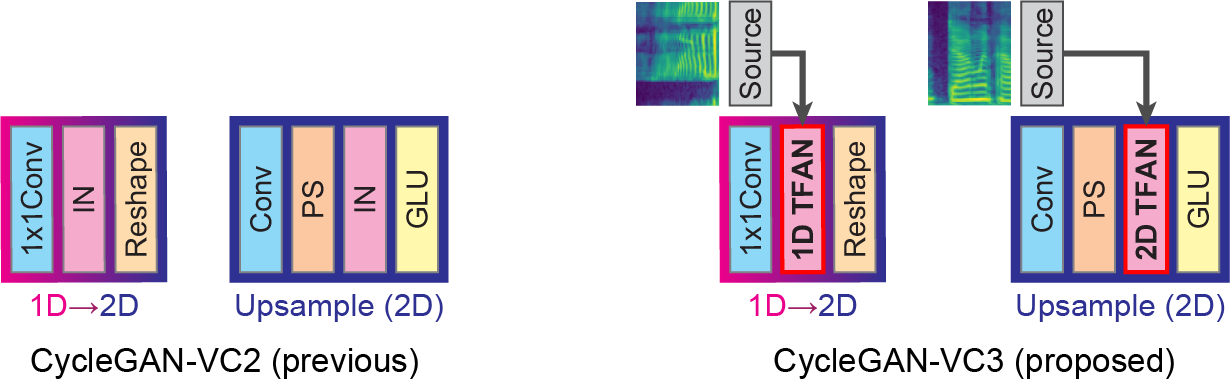
Conversion samples
Recommended browsers: Safari, Chrome, Firefox, and Opera.
Experimental conditions
- We evaluated our method on the Spoke (i.e., non-parallel VC) task of the Voice Conversion Challenge 2018 (VCC 2018) [4].
- For each speaker, 81 utterances (approximately 5 minutes; which is relatively low for VC) were used for training.
- In the training set, there are no overlaps between source and target utterances; therefore, this problem must be solved in a fully non-parallel setting.
- We did not use any extra data, module, or time alignment procedures for training.
Compared models
- B: Mel-cepstrum conversion by CycleGAN-VC2 [2] + WORLD vocoder [5].
- V1: Mel-spectrogram conversion by CycleGAN-VC [1] + MelGAN vocoder [6].
- V2: Mel-spectrogram conversion by CycleGAN-VC2 [2] + MelGAN vocoder [6].
- V3: Mel-spectrogram conversion by CycleGAN-VC3 + MelGAN vocoder [6].
Results
- Female (VCC2SF3) → Female (VCC2TF1)
- Male (VCC2SM3) → Male (VCC2TM1)
- Female (VCC2SF3) → Male (VCC2TM1)
- Male (VCC2SM3) → Female (VCC2TF1)
Female (VCC2SF3) → Female (VCC2TF1)
Source | Target | B | V1 | V2 | V3 | |
---|---|---|---|---|---|---|
Sample 1 | ||||||
Sample 2 | ||||||
Sample 3 |
Male (VCC2SM3) → Male (VCC2TM1)
Source | Target | B | V1 | V2 | v3 | |
---|---|---|---|---|---|---|
Sample 1 | ||||||
Sample 2 | ||||||
Sample 3 |
Female (VCC2SF3) → Male (VCC2TM1)
Source | Target | B | V1 | V2 | V3 | |
---|---|---|---|---|---|---|
Sample 1 | ||||||
Sample 2 | ||||||
Sample 3 |
Male (VCC2SM3) → Female (VCC2TF1)
Source | Target | B | V1 | V2 | V3 | |
---|---|---|---|---|---|---|
Sample 1 | ||||||
Sample 2 | ||||||
Sample 3 |