A paper accepted for publication in the IEEE Transactions on Audio, Speech and Language Processing
SoundBeam: Target Sound Extraction Conditioned on Sound-class Labels and Enrollment Clues for Increased Performance and Continuous Learning
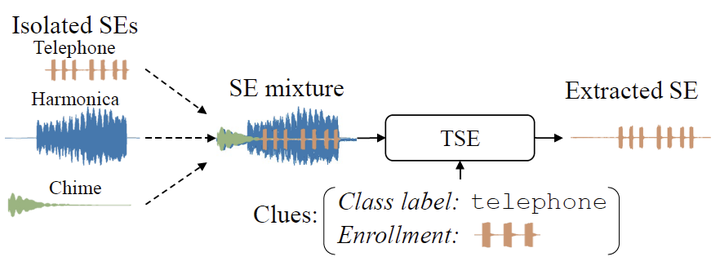
We are pleased to announce that our paper "SoundBeam: Target Sound Extraction Conditioned on Sound-class Labels and Enrollment Clues for Increased Performance and Continuous Learning" by Marc Delcroix, Jorge Bennasar Vázquez, Tsubasa Ochiai, Keisuke Kinoshita, Yasunori Ohishi, and Shoko Araki has been accepted for publication in the IEEE Transactions on Audio, Speech and Language Processing.
This paper provides an unified description of the class label and enrollment-based target sound extraction (TSE), and introduce SoundBeam, which is a TSE model that combines both class label- and enrollment-based approaches. SoundBeam learns an embedding space common to class label and enrollment-based target SE embeddings by sharing the extraction NN. Consequently, we can thus use optimal SE embeddings for known SE classes with the class label embeddings and generalize to new SE classes with the enrollment embeddings. Besides, we show that SoundBeam has potential for continuous learning as it can learn new classes with few-shot adaptation.