09 |
AI that attends to the sounds you want to listen toDeep learning based selective hearing of arbitrary sounds ![]() |
---|
Humans can focus on listening to a desired sound in complex sound scenes consisting of many co-occurring sounds to retrieve important information, an ability known as selective hearing. In this work, we introduce SoundBeam, a deep-learning-based approach for computational selective hearing. SoundBeam extends our prior work on selective hearing of speech based on the characteristics of the target speaker’s voice to arbitrary sounds. With SoundBeam, users can control which sound to listen to depending on their preference or the environment, e.g., hearing a klaxon when crossing a street but not when working at home, thus creating comfortable and safe sound environments.
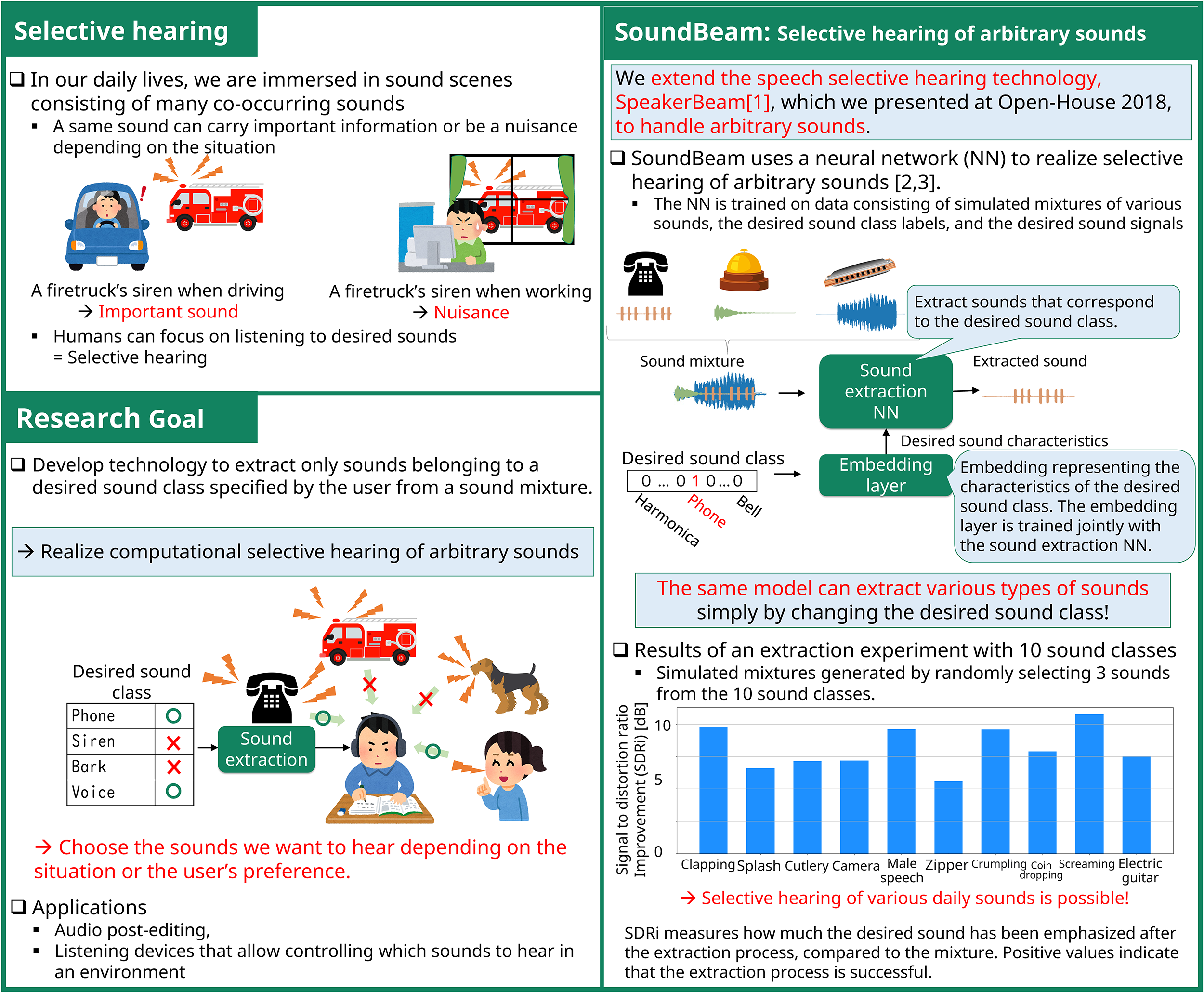
[1] M. Delcroix, K. Zmolikova, K. Kinoshita, S. Araki, A. Ogawa, and T. Nakatani, “SpeakerBeam: A New Deep Learning Technology for Extracting Speech of a Target Speaker Based on the Speaker’s Voice Characteristics,” NTT Technical Review, Vol. 16, No. 11, pp. 19–24, Nov. 2018.
[2] T. Ochiai, M. Delcroix, Y. Koizumi, H. Ito, K. Kinoshita, S. Araki, “Listen to what you want: Neural network-based universal sound selector,” in Proc. Interspeech, pp. 2718 - 2722, 2020.
[3] M. Delcroix, J. B. Vázquez, T. Ochiai, K. Kinoshita, Y. Ohishi, S. Araki, "SoundBeam: Target Sound Extraction Conditioned on Sound-Class Labels and Enrollment Clues for Increased Performance and Continuous Learning," IEEE/ACM Trans. on Audio, Speech, and Language Processing, Vol. 31, pp. 121-136, 2023.
Marc Delcroix
Signal Processing Research Group, Media Information Laboratory