Please check out our relevant work!
Follow-up work: Previous work: Other relevant work:- StarGAN-VCs: StarGAN-VC, StarGAN-VC2
- Other VCs: Links to demo pages
Paper
Takuhiro Kaneko, Hirokazu Kameoka, Kou Tanaka, and Nobukatsu Hojo
CycleGAN-VC2: Improved CycleGAN-based Non-parallel Voice Conversion
ICASSP 2019
(arXiv:1904.04631, Apr. 2019)
[Paper]
[IEEE Xplore]
[Poster]
[BibTeX]
CycleGAN-VC2
To advance the research on non-parallel VC, we propose CycleGAN-VC2, which is an improved version of CycleGAN-VC incorporating three new techniques: an improved objective (two-step adversarial losses), improved generator (2-1-2D CNN), and improved discriminator (Patch GAN).
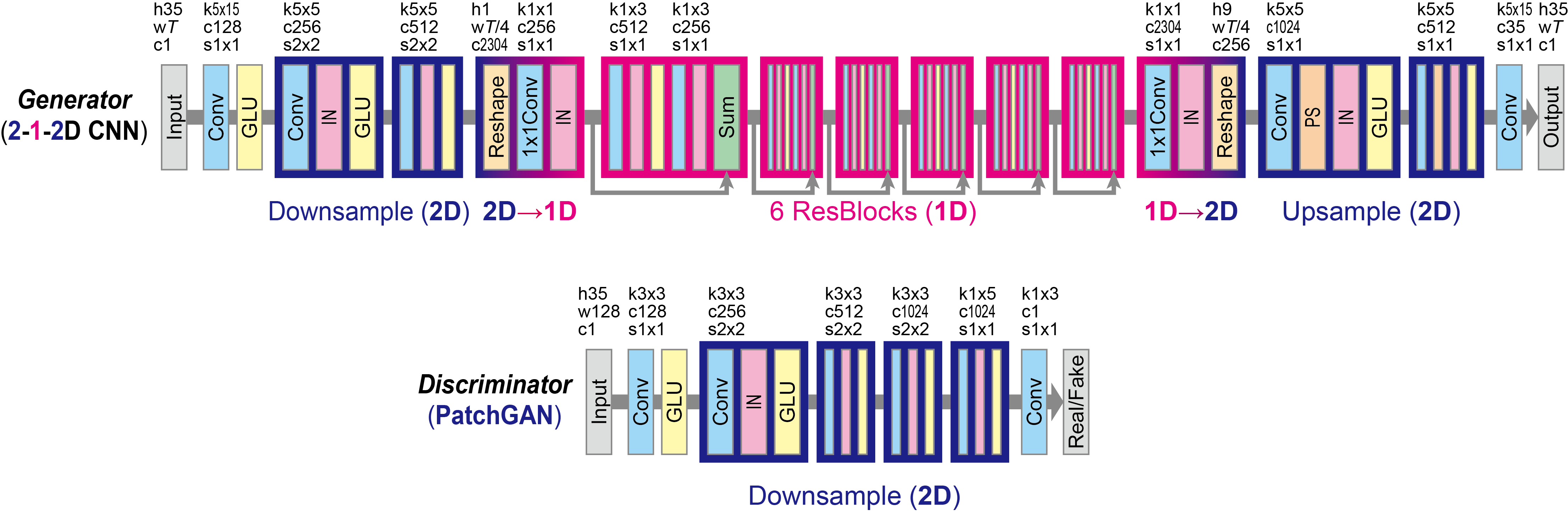
Converted speech samples
Experimental conditions
Dataset
- We evaluated our method on the Spoke (i.e., non-parallel VC) task of the Voice Conversion Challenge 2018 (VCC 2018) dataset [1].
- Each speaker has 81 sentences (about 5 minutes) for training. This is relatively little for VC.
- The sentence set of the source speakers is different (no overlap) from that of the target speakers so as to evaluate in a non-parallel setting.
- Note that we did not use any extra data, module, or time alignment procedures.
Voice conversion framework
- In intra-gender conversion, a vocder-free VC framework [2] was used. The converted speech is generated by filtering the source speech by using differential MCEPs. This is the same as the VCC 2018 baseline [3].
- In inter-gender conversion, a vocder-based VC framework [4] was used. The converted speech is generated by using the WORLD synthesizer [5] with converted acoustic features. This is the same as the VCC 2018 baseline [3].
Notation
- Source is the source speech samples.
- Target is the target speech samples. They are provided as references. Note that we did not use such paired data in training.
- CycleGAN-VC is the converted speech samples, in which the conventional CycleGAN-VC [6] was used to convert MCEPs.
- CycleGAN-VC2 is the converted speech samples, in which the proposed CycleGAN-VC2 was used to convert MCEPs.
- CycleGAN-VC2++ is the converted speech samples, in which the proposed CycleGAN-VC2 was used to convert all acoustic features (namely, MCEPs, band APs, continuous log F0, and voice/unvoice indicator). When using a vocoder-free VC framework, all acoustic features were used for training, but only MCEPs were used for conversion.
Results
NOTE: Recommended browsers are Apple Safari, Google Chrome, or Mozilla Firefox.
Intra-gender conversion (vocoder-free VC [2])
Female (VCC2SF3) → Female (VCC2TF1)
Source | Target | CycleGAN-VC (Conventional) |
CycleGAN-VC2 (Proposed) |
CycleGAN-VC2++ (Proposed) |
|
---|---|---|---|---|---|
Sample 1 | |||||
Sample 2 | |||||
Sample 3 |
Male (VCC2SM3) → Male (VCC2TM1)
Source | Target | CycleGAN-VC (Conventional) |
CycleGAN-VC2 (Proposed) |
CycleGAN-VC2++ (Proposed) |
|
---|---|---|---|---|---|
Sample 1 | |||||
Sample 2 | |||||
Sample 3 |
Inter-gender conversion (vocoder-based VC [4])
Male (VCC2SM3) → Female (VCC2TF1)
Source | Target | CycleGAN-VC (Conventional) |
CycleGAN-VC2 (Proposed) |
CycleGAN-VC2++ (Proposed) |
|
---|---|---|---|---|---|
Sample 1 | |||||
Sample 2 | |||||
Sample 3 |
Female (VCC2SF3) → Male (VCC2TM1)
Source | Target | CycleGAN-VC (Conventional) |
CycleGAN-VC2 (Proposed) |
CycleGAN-VC2++ (Proposed) |
|
---|---|---|---|---|---|
Sample 1 | |||||
Sample 2 | |||||
Sample 3 |
References
[1] J. Lorenzo-Trueba, J. Yamagishi, T. Toda, D. Saito, F. Villavicencio, T. Kinnunen, and Z. Ling. The Voice Conversion Challenge 2018: Promoting Development of Parallel and Nonparallel Methods. Odyssey, 2018. [Paper] [Dataset]
[2] K. Kobayashi, T. Toda, and S. Nakamura. F0 Transformation Techniques for Statistical Voice Conversion with Direct Waveform Modification with Spectral Differential. SLT, 2016. [Paper] [Project]
[3] K. Kobayashi and T. Toda. sprocket: Open-Source Voice Conversion Software. Odyssey, 2018. [Paper] [Project]
[4] T. Toda, A. W Black, and K. Tokuda. Voice Conversion Based on Maximum Likelihood Estimation of Spectral Parameter Trajectory. IEEE Trans. Audio Speech Lang. Process., 2007. [Paper]
[5] M. Morise, F. Yokomori, and K. Ozawa. WORLD: A Vocoder-Based High-Quality Speech Synthesis System for Real-Time Applications. IEICE Trans. Inf. Syst., 2016. [Paper] [Project]
[6] T. Kaneko and H. Kameoka. Parallel-Data-Free Voice Conversion Using Cycle-Consistent Adversarial Networks. arXiv:1711.11293, Nov. 2017 (EUSIPCO, 2018). [Paper] [Project]
[7] H. Kameoka, T. Kaneko, K. Tanaka, and N. Hojo. StarGAN-VC: Non-parallel Many-to-Many Voice Conversion with Star Generative Adversarial Networks. SLT, 2018. [Paper] [Project]
[8] T. Kaneko, H. Kameoka, K. Tanaka, and N. Hojo. StarGAN-VC2: Rethinking Conditional Methods for StarGAN-Based Voice Conversion. Interspeech, 2019. [Paper] [Project]
[9] H. Kameoka, T. Kaneko, K. Tanaka, and N. Hojo. ACVAE-VC: Non-parallel Voice Conversion with Auxiliary Classifier Variational Autoencoder. IEEE/ACM Trans. Audio Speech Lang. Process., May 2019. [Paper] [Project]
[10] C.-C. Hsu, H.-T. Hwang, Y.-C. Wu, Y. Tsao, and H.-M. Wang. Voice Conversion from Unaligned Corpora using Variational Autoencoding Wasserstein Generative Adversarial Networks. Interspeech, 2017. [Paper] [Project]