Please check out our relevant work!
Previous work:- CycleGAN-VCs: CycleGAN-VC, CycleGAN-VC2, CycleGAN-VC3,
- StarGAN-VCs: StarGAN-VC, StarGAN-VC2
- Other VCs: Links to demo pages
MaskCycleGAN-VC
Non-parallel voice conversion (VC) is a technique for training voice converters without a parallel corpus. Cycle-consistent adversarial network-based VCs (CycleGAN-VC [1] and CycleGAN-VC2 [2]) are widely accepted as benchmark methods. However, owing to their insufficient ability to grasp time-frequency structures, their application is limited to mel-cepstrum conversion and not mel-spectrogram conversion despite recent advances in mel-spectrogram vocoders. To overcome this, CycleGAN-VC3 [3], an improved variant of CycleGAN-VC2 that incorporates an additional module called time-frequency adaptive normalization (TFAN), has been proposed. However, an increase in the number of learned parameters is imposed. As an alternative, we propose MaskCycleGAN-VC, which is another extension of CycleGAN-VC2 and is trained using a novel auxiliary task called filling in frames (FIF). With FIF, we apply a temporal mask to the input mel-spectrogram and encourage the converter to fill in missing frames based on surrounding frames. This task allows the converter to learn time-frequency structures in a self-supervised manner and eliminates the need for an additional module such as TFAN. A subjective evaluation of the naturalness and speaker similarity showed that MaskCycleGAN-VC outperformed both CycleGAN-VC2 and CycleGAN-VC3 with a model size similar to that of CycleGAN-VC2.
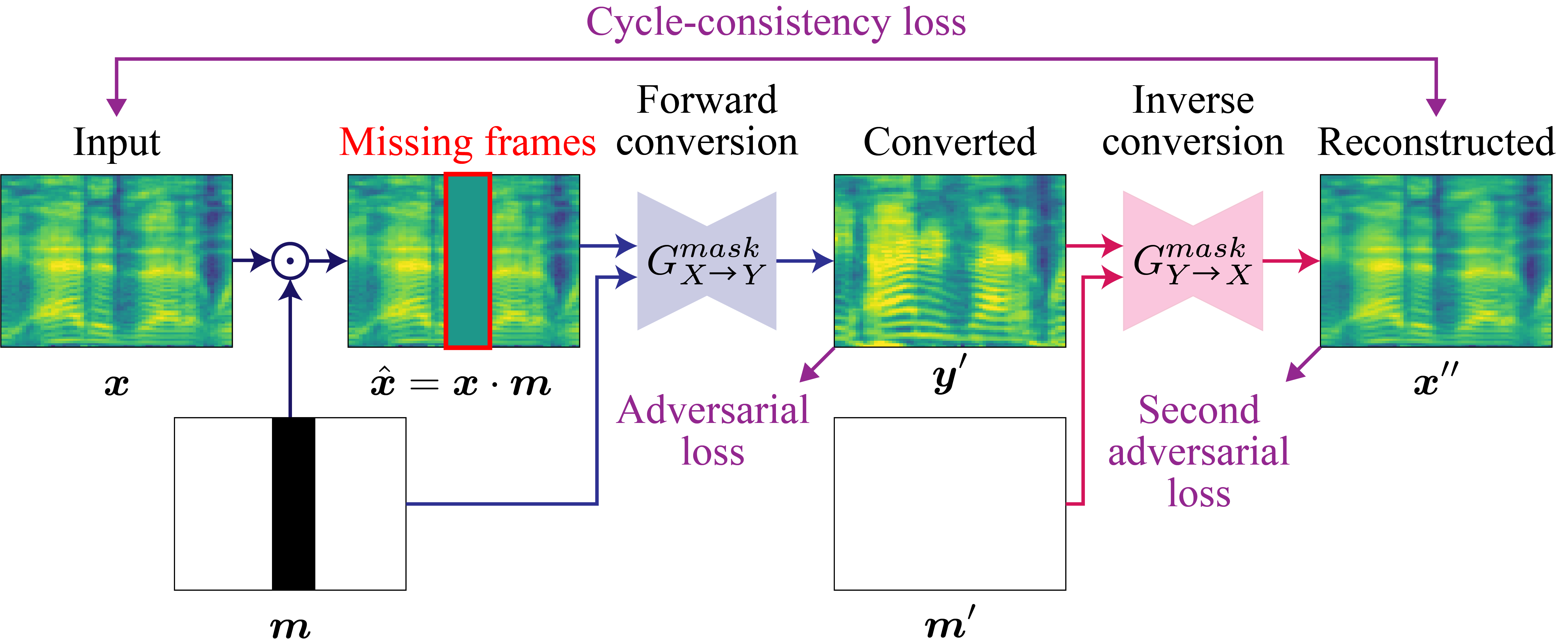
Conversion samples
Recommended browsers: Safari, Chrome, Firefox, and Opera.
Experimental conditions
- We evaluated our method on the Spoke (i.e., non-parallel VC) task of the Voice Conversion Challenge 2018 (VCC 2018) [4].
- For each speaker, 81 sentences (approximately 5 min in length, which is relatively short for VC) were used for training.
- The training set contains no overlapping utterances between the source and target speakers; therefore, we need to learn a converter in a fully non-parallel setting.
- We used MelGAN [5] as a vocoder.
Compared models
- V2: CycleGAN-VC2 [2]
- V3: CycleGAN-VC3 [3]
- Mask: MaskCycleGAN-VC
Results
- Female (VCC2SF3) → Male (VCC2TM1)
- Male (VCC2SM3) → Female (VCC2TF1)
- Female (VCC2SF3) → Female (VCC2TF1)
- Male (VCC2SM3) → Male (VCC2TM1)
Female (VCC2SF3) → Male (VCC2TM1)
Source | Target | V2 | V3 | Mask | |
---|---|---|---|---|---|
Sample 1 | |||||
Sample 2 | |||||
Sample 3 |
Male (VCC2SM3) → Female (VCC2TF1)
Source | Target | V2 | V3 | Mask | |
---|---|---|---|---|---|
Sample 1 | |||||
Sample 2 | |||||
Sample 3 |
Female (VCC2SF3) → Female (VCC2TF1)
Source | Target | V2 | V3 | Mask | |
---|---|---|---|---|---|
Sample 1 | |||||
Sample 2 | |||||
Sample 3 |
Male (VCC2SM3) → Male (VCC2TM1)
Source | Target | V2 | v3 | Mask | |
---|---|---|---|---|---|
Sample 1 | |||||
Sample 2 | |||||
Sample 3 |