A paper presented at ICASSP 2023
Masked Modeling DUO: Learning Representations by Encouraging both Networks to Model the Input
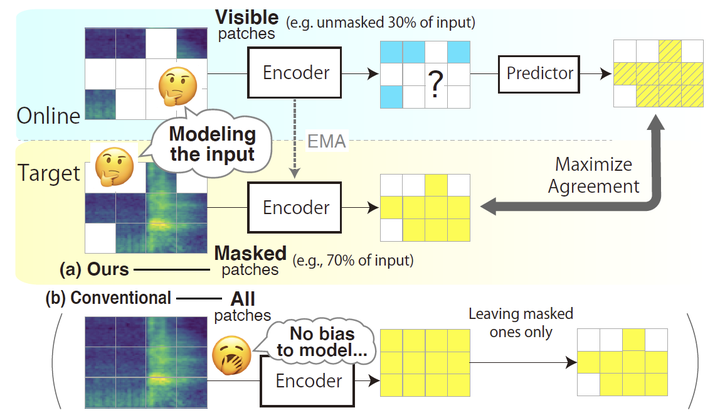
We are pleased to announce that our paper "Masked Modeling DUO: Learning Representations by Encouraging both Networks to Model the Input" by Daisuke Niizumi, Daiki Takeuchi, Yasunori Ohishi, Noboru Harada, and Kunio Kashino has been accepted to ICASSP 2023.
This paper proposes a new method, Masked Modeling Duo (M2D), that learns representations directly while obtaining training signals using only masked patches. In the M2D, the online network encodes visible patches and predicts masked patch representations, and the target network, a momentum encoder, encodes masked patches. To better predict target representations, the online network should model the input well, while the target network should also model it well to agree with online predictions. Then the learned representations should better model the input. We validated the M2D by learning general-purpose audio representations, and M2D set new state-of-the-art performance on tasks such as UrbanSound8K, VoxCeleb1, AudioSet20K, GTZAN, and SpeechCommandsV2.