01 |
Learning how to learn from various datasetsMeta-learning from tasks with heterogeneous attribute spaces ![]() |
---|
Many training data are required for deep learning. We propose a meta-learning method that can improve the performance with a limited number of training data in the target task by using data in different tasks. For example, the proposed method can be used for learning from data in different factories. Existing meta-learning methods assumes that all tasks have the same attribute space. The proposed method is the first method that can meta-learn from tasks with different attribute spaces. With the proposed method, we design permutation invariant neural networks that can handle data with different attributes and labels. Currently, we cannot apply machine learning methods when sufficient number of training data are unavailable. We want to expand field where machine learning methods are applicable by extending the proposed method.
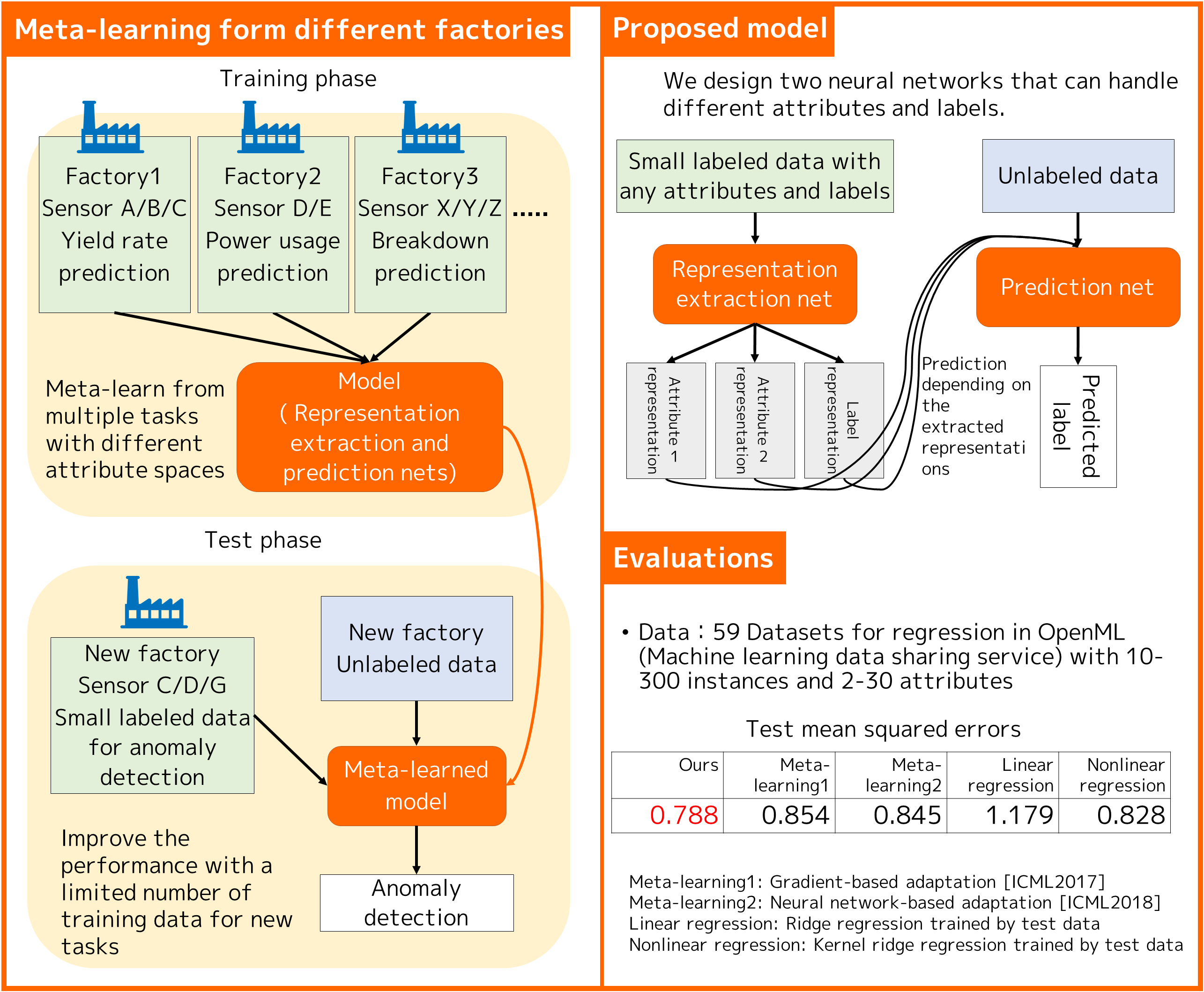
[1] T. Iwata, A. Kumagai, “Meta-learning from Tasks with Heterogeneous Attribute Spaces,” in Proc. Neural Information Processing Systems (NeurIPS), 2020.
Tomoharu Iwata / Ueda Research Laboratory
Email: cs-openhouse-ml@hco.ntt.co.jp