04 |
No labels? Count on me!Self-supervised adaptation for unknown domains/classes ![]() |
---|
We propose a method that enables us to use miscellaneous data collected under various conditions directly for deep learning. When we train a model using miscellaneous data, the model's performance is often degraded by "Information to be Ignored," which disturbs correct recognition. Our proposed method dramatically improves the model's performance by estimating the "Information to be Ignored" and training the model not to be affected by it. Our technique will make it possible to easily utilize miscellaneous data for learning and will contribute to expanding AI services into fields where deploying deep learning has been challenging.
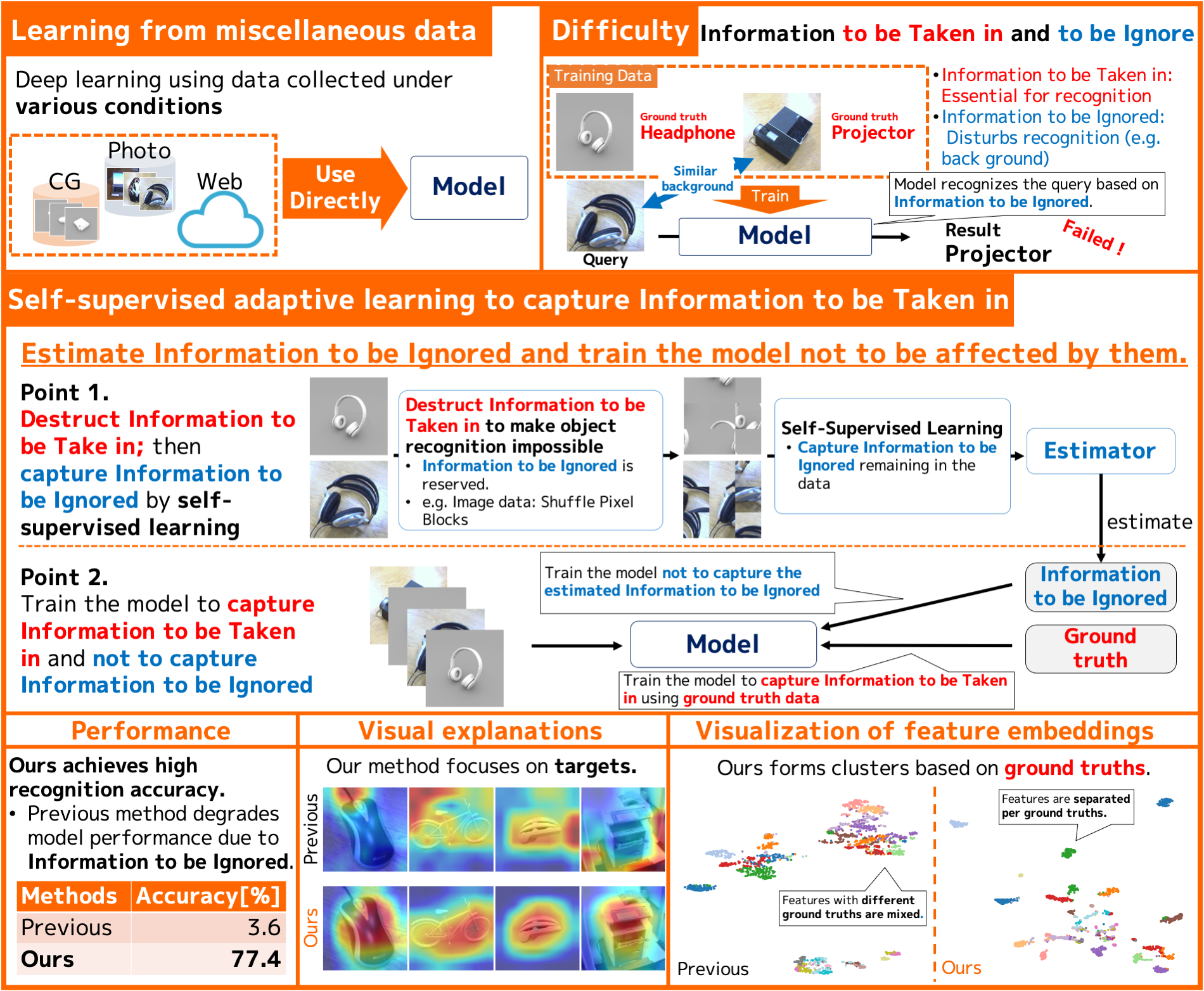
[1] Y. Mitsuzumi, G. Irie, D. Ikami, T. Shibata, “Generalized Domain Adaptation,” in Proc. IEEE/CVF Conference on Computer Vision and Pattern Recognition (CVPR), 2021.
[2] R. Tobias, R. Stiefelhagen, “Adaptiope: A Modern Benchmark for Unsupervised Domain Adaptation,” in Proc. IEEE/CVF Winter Conference on Applications of Computer Vision (WACV), 2021.
Yu Mitsuzumi / Recognition Research Group, Media Information Laboratory
Email: cs-openhouse-ml@hco.ntt.co.jp