03 |
Machine learning that reproduces physical phenomenaGaussian process model incorporating energy conservation law ![]() |
---|
Our research question aims to reproduce the underlying behavior that adheres to the laws of physics using machine learning techniques. We propose a novel Gaussian process model that incorporates the theory of Hamiltonian mechanics. One advantage of our technology is that it can simulate physical phenomena without handcrafted equations. Our experiments show that our technology accurately simulates physical phenomena that follow energy conservation laws even in noisy and small data scenarios. This research enables us to automatically construct simulators for complex physical phenomena from observed data. We expect our research to contribute to the development of such science/industries as weather forecasting as well as improving the efficiency and the quality of aircraft design.
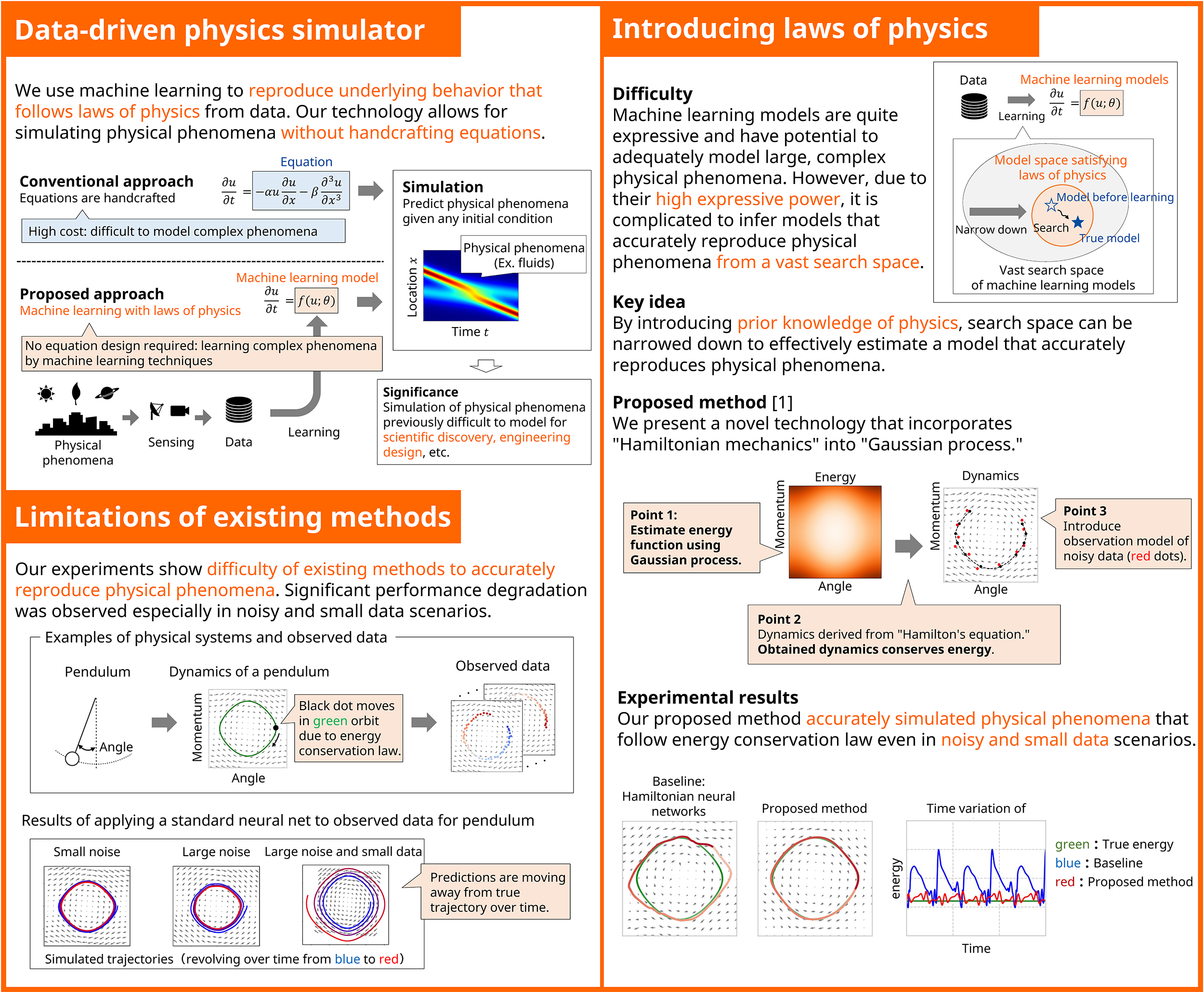
[1] Y. Tanaka, T. Iwata, N. Ueda, “Symplectic spectrum Gaussian processes: Learning Hamiltonians from noisy and sparse data,” in Proc. Advances in Neural Information Processing Systems (NeurIPS), 2022.
Yusuke Tanaka
Learning and Intelligent Systems Research Group, Innovative Communication Laboratory