02 |
Efficient training of photonic AIAccelerated learning of fine-layered optical neural networks ![]() |
---|
An optical neural network (ONN) is a promising system due to its high-speed and low-power operation. The ONN has a multiple-layered structure of programmable Mach-Zehnder interferometers (MZIs). Due to this structure, it takes a lot of time to learn MZI parameters with a conventional automatic differentiation (AD). To solve the time-consuming problem, we develop a function module implemented in C++ to collectively calculate input-output values in a multiple-layered structure, where novel customized derivatives for an MZI are utilized in backpropagation. We demonstrate that our learning method works 50 times faster than the conventional AD when a pixel-by-pixel MNIST task is performed in a complex-valued recurrent neural network. Our approach supports ONN design and contributes to realize green-computing AI's instead of conventional ones consuming a lot of energy.
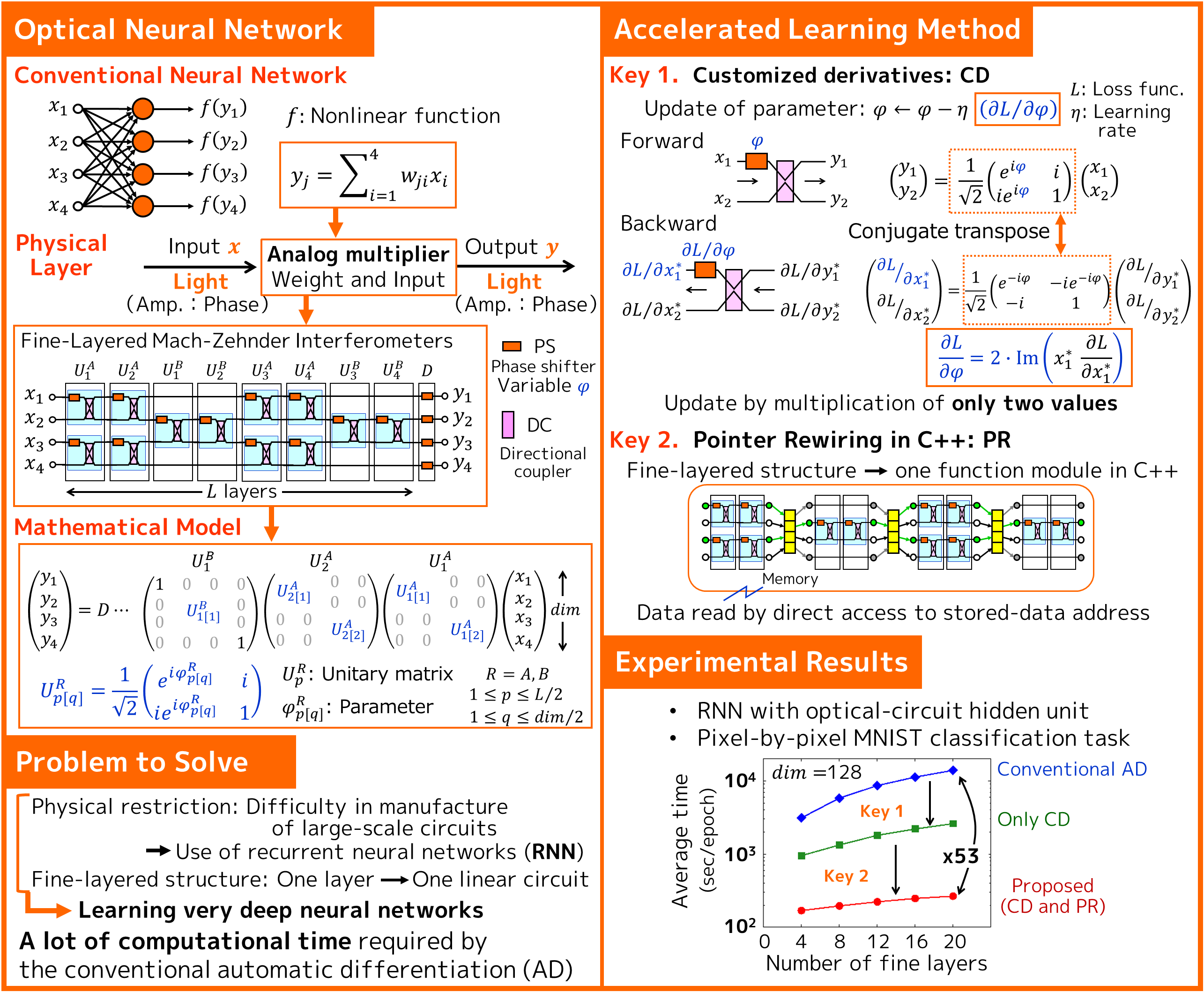
[1] K. Aoyama, H. Sawada, “Accelerated method for learning fine-layered optical neural networks,” in Proc. of IEEE/ACM the 40th International Conference on Computer-Aided Design, 2021.
Kazuo Aoyama / Learning and Intelligent Systems Research Group, Innovative Communication Laboratory
Email: cs-openhouse-ml@hco.ntt.co.jp