15 |
Flexible bokeh renderer based on predicted depthDeep generative model for learning depth and bokeh effects only from natural images ![]() |
---|
Based on their experience and knowledge, humans can estimate depth and bokeh effects from the corresponding 2D images. However, computers have difficulty in doing so because they lack the necessary experience and knowledge. To overcome this limitation, we propose a novel deep generative model that can control bokeh effects based on predicted depth. If it is possible to collect pairs of 2D images and 3D information, learning a 3D predictor is simple because of direct supervision. However, collecting such data is often difficult or impractical owing to the requirement for specific sensors, such as a depth sensor or stereo camera. To eliminate this requirement, we developed the world's first technology that enables learning depth and bokeh effects only from standard 2D images. Because we live in a 3D world, a human-oriented computer must understand the 3D world. This study addresses this challenge by eliminating an application boundary in terms of data collection cost. We expect that this technology will cultivate a new field of 3D understanding.
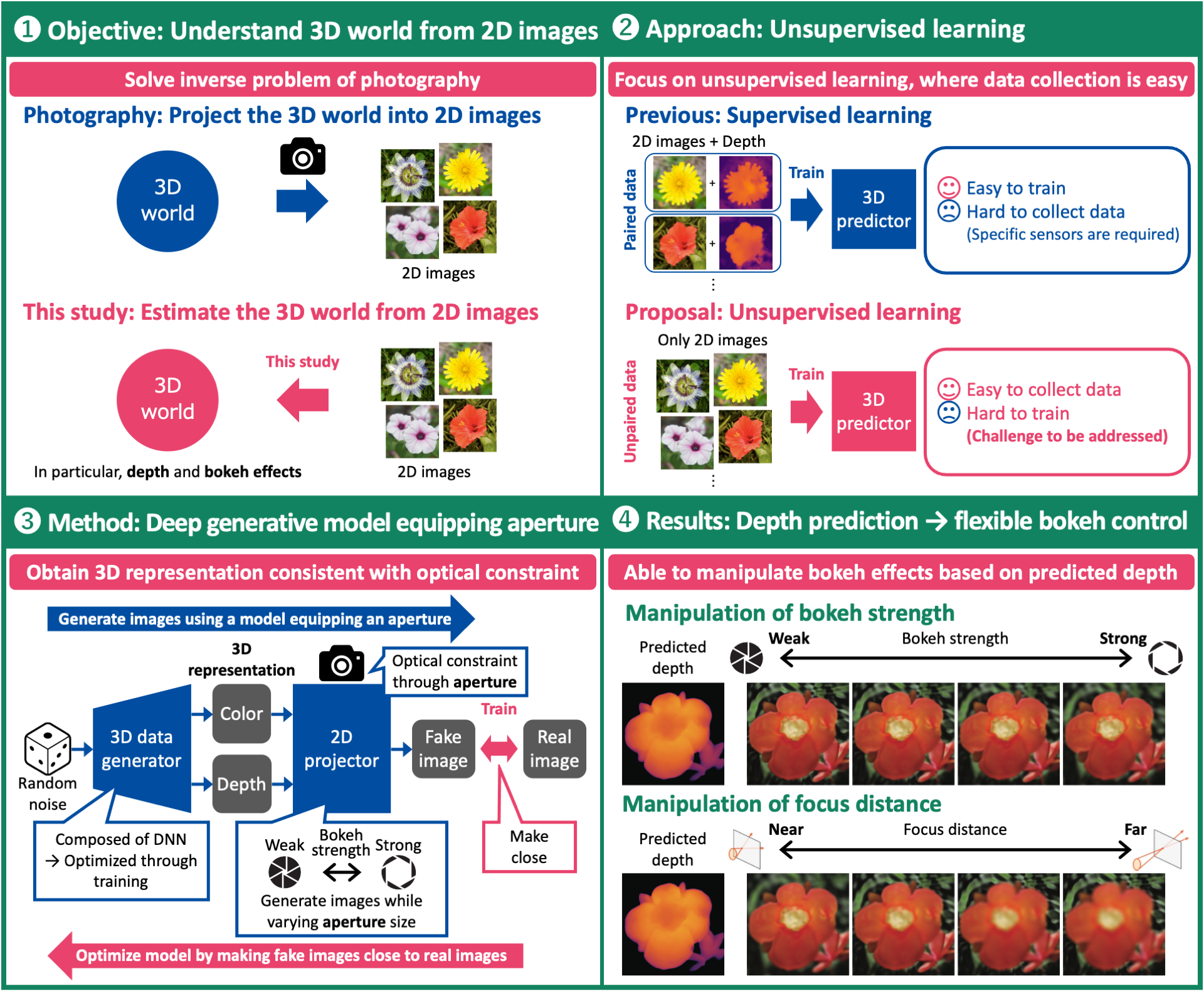
[1] T. Kaneko, “Unsupervised learning of depth and depth-of-field effect from natural images with aperture rendering generative adversarial networks,” in Proc. 2021 IEEE/CVF Conference on Computer Vision and Pattern Recognition (CVPR2021), pp. 15679–15688, 2021.
[2] T. Kaneko, “AR-NeRF: Unsupervised learning of depth and defocus effects from natural images with aperture rendering neural radiance fields,” in Proc. 2022 IEEE/CVF Conference on Computer Vision and Pattern Recognition (CVPR2022), 2022 (to appear).
Takuhiro Kaneko / Recognition Research Group, Media Information Laboratory
Email: cs-openhouse-ml@hco.ntt.co.jp