03 |
Multiple AIs make better predictionsBayesian ensemble learning for better generalization performance ![]() |
---|
Evaluating the certainty of the prediction is essential for machine learning tasks. For example, certainty is required to assess the predictions' reliability, decision-making, and experimental design problems. We developed a method to efficiently calculate the certainty for a large model such as a neural network using an ensemble of models. Although evaluating the certainty of predictions using an ensemble of models has been widely used in existing work, it was theoretically unclear how to prepare ensembles. Our research theoretically derived an algorithm for preparing ensembles for expressing the certainty of prediction using multiple models. Evaluating the uncertainty is important to make machine learning reliable. We can easily evaluate the certainty using an ensemble of models and expand the range of machine learning applications by proceeding with this research.
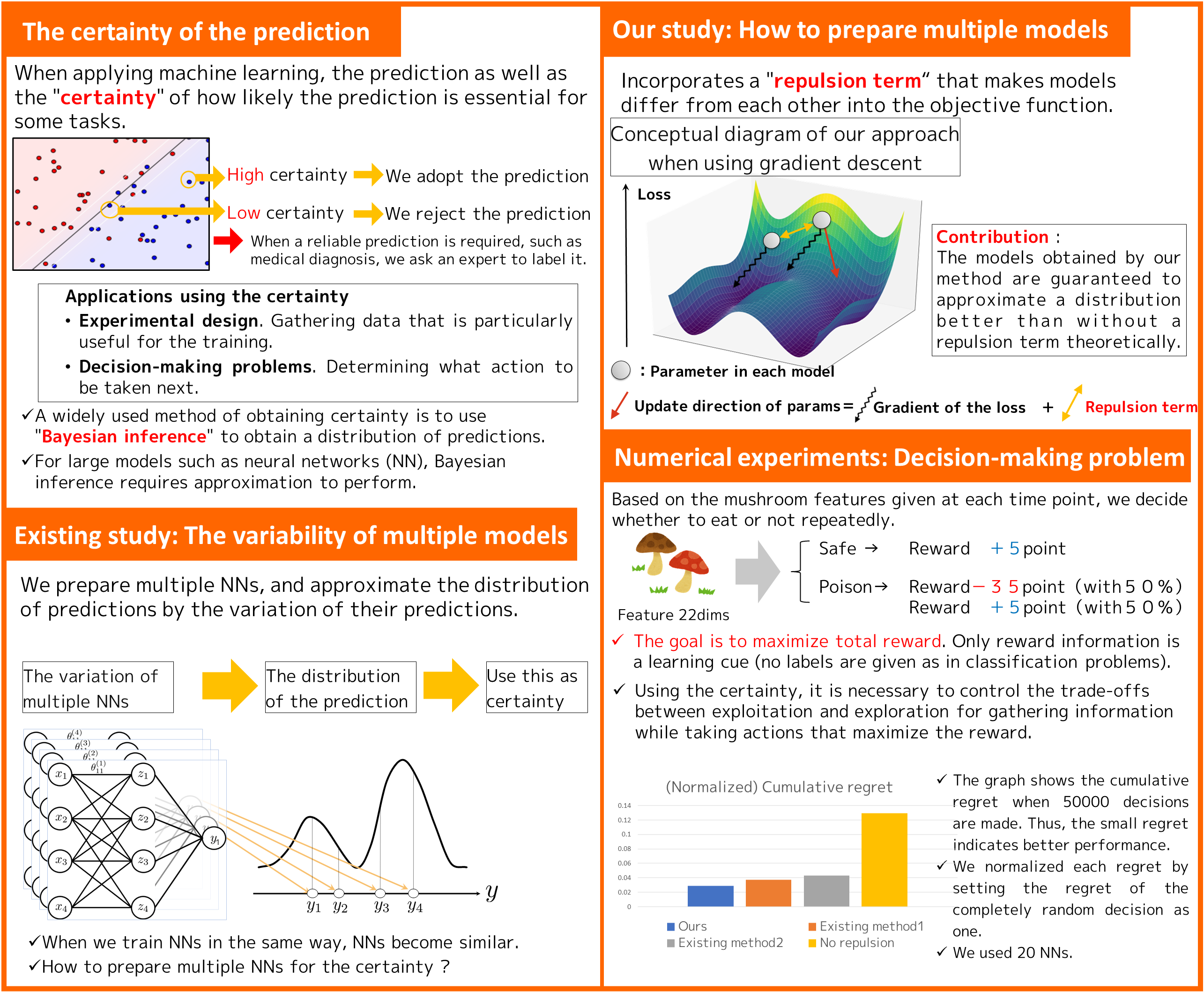
[1] F. Futami, T. Iwata, N. Ueda, I. Sato, M. Sugiyama, “Loss function based second-order Jensen inequality and its application to particle variational inference,” in Proc. Neural Information Processing Systems (NeurIPS), 2021.
Futami Futoshi / Ueda Research Laboratory
Email: cs-openhouse-ml@hco.ntt.co.jp