18 |
Communication-efficient learning over data centersFinite-time convergent graph for fast decentralized learning ![]() |
---|
Decentralized learning is a fundamental technology to efficiently train machine learning models from a large amount of data by using computing nodes connected over a network (graph). Our proposed Base (k+1) graph guarantees finite-time convergence with any number of nodes and maximum number of connections (degree), enabling fast and stable decentralized learning. We evaluated the efficiency of the graph in a situation where each node has a statistically heterogeneous data subset, and confirmed that it can achieve fast and stable learning of models. This technology, which satisfies finite-time convergence while minimizing the number of operations and communications, will lead to reduce the entire power consumption of data centers.
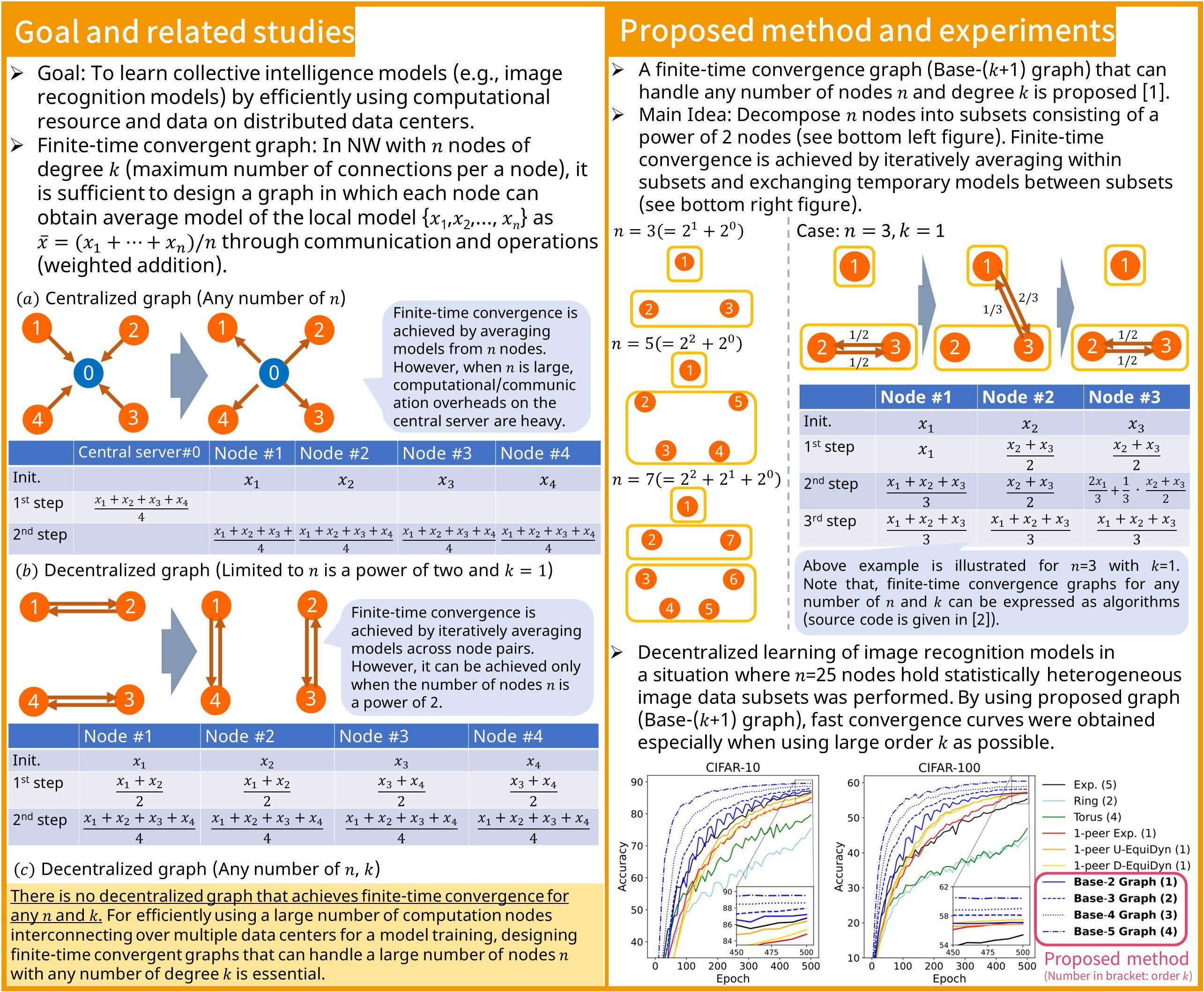
[1] Y. Takezawa, R. Sato, H. Bao, K. Niwa, and M. Yamada, “Beyond exponential graph: communication-efficient topologies for decentralized learning via finite-time convergence”, in Proc. The Thirty-seventh Annual Conference on Neural Information Processing Systems (NeurIPS2023), 2023.
Kenta Niwa, Learning and Intelligent Systems Research Group, Innovative Communication Laboratory