01 |
Collaborative learning with multiple thoughtsTransparent prediction based on decision tree superposition ![]() |
---|
Decision trees have been playing an important role in machine learning tasks that require interpretability and transparency, such as in finance and biomedical fields. In recent years, the existence of multiple decision tree candidates with comparable performance but different qualities, known as the Rashomon set, has attracted attention as a topic that further promotes the interpretability and transparency of decision trees. In this presentation, we explore a method for exploring the Rashomon set for decision trees. Specifically, we propose a quantum extension of decision trees in order to introduce quantum effects as a mechanism for multiple influential decision trees of seemingly different quality in a Rashomon set to share potentially useful clues with each other.
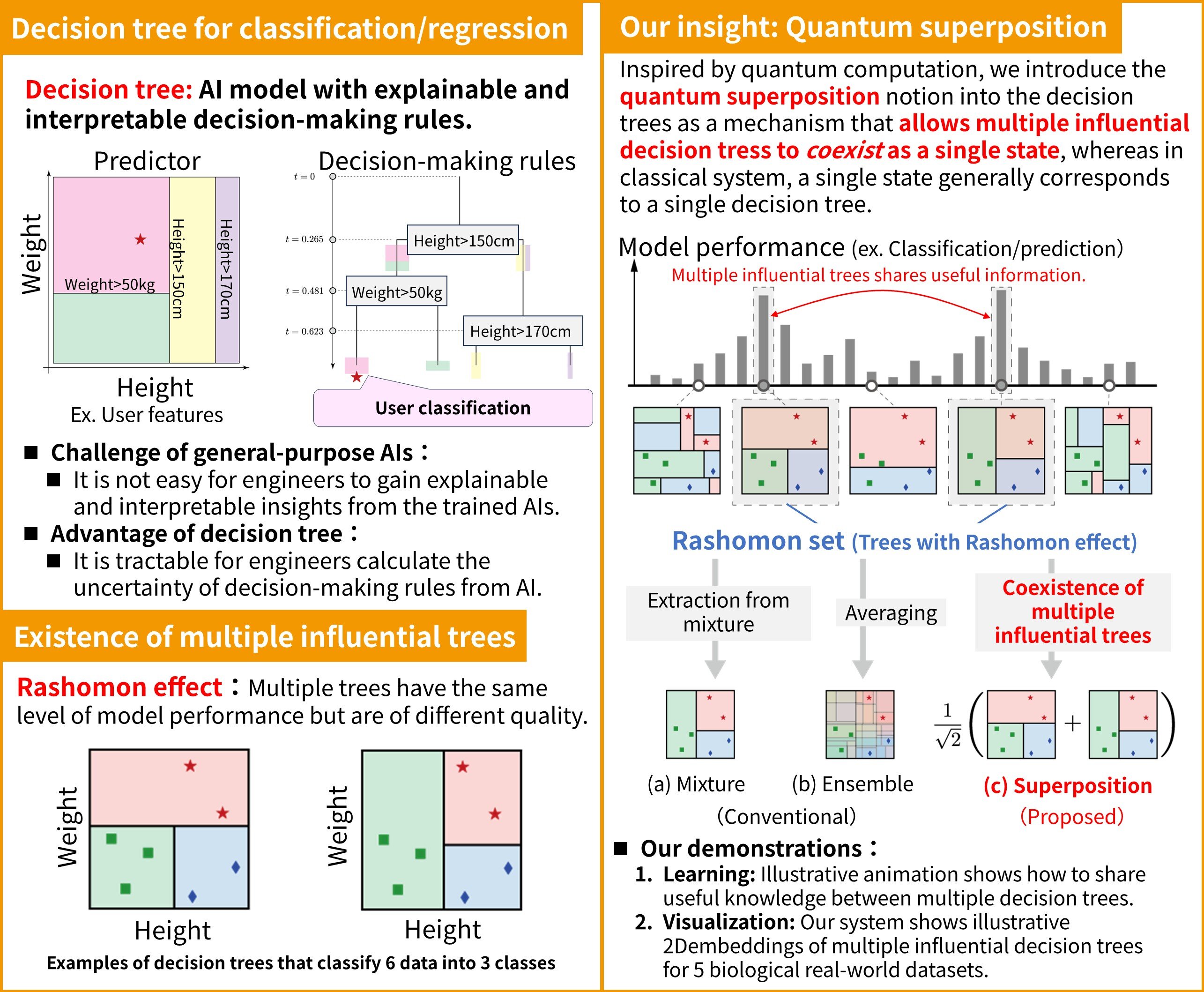
[1] M. Nakano, K. Komiya, H. Sakuma, T. Sato, T. Iwata, K. Kashino, “Mondrian Embeddings for Visualization of Decision Tree Ensembles,” in Proc. the 47th annual international conference of the IEEE Engineering in Medicine and Biology Society.
Masahiro Nakano, Biomedical Informatics Research Group, Media Information Laboratory