04 |
Toward the realization of low-power optical AITraining of optical neural networks with special structure ![]() |
---|
Optical neural networks (ONNs) are expected to operate with ultra-low power consumption. Unlike neural networks implemented on conventional computers, ONNs are directly implemented in hardware, whose internal information cannot be easily observed. Thus, the popular back-propagation algorithm, which requires the detailed internal information, cannot be used to train ONNs. We study a black-box optimization method that trains neural networks by observing the input-output relationship from outside the hardware. In this study, we point out that the special structure of ONNs causes a problem of multiple parameters being interrelated. We propose a new method that solves the problem and trains ONNs effectively. With the rapid progress of artificial intelligence, represented by generative AI, a huge amount of electricity is consumed on a global scale. By realizing ONNs, we aim for a carbon-neutral future in which artificial intelligence can be used in every situation with less power consumption.
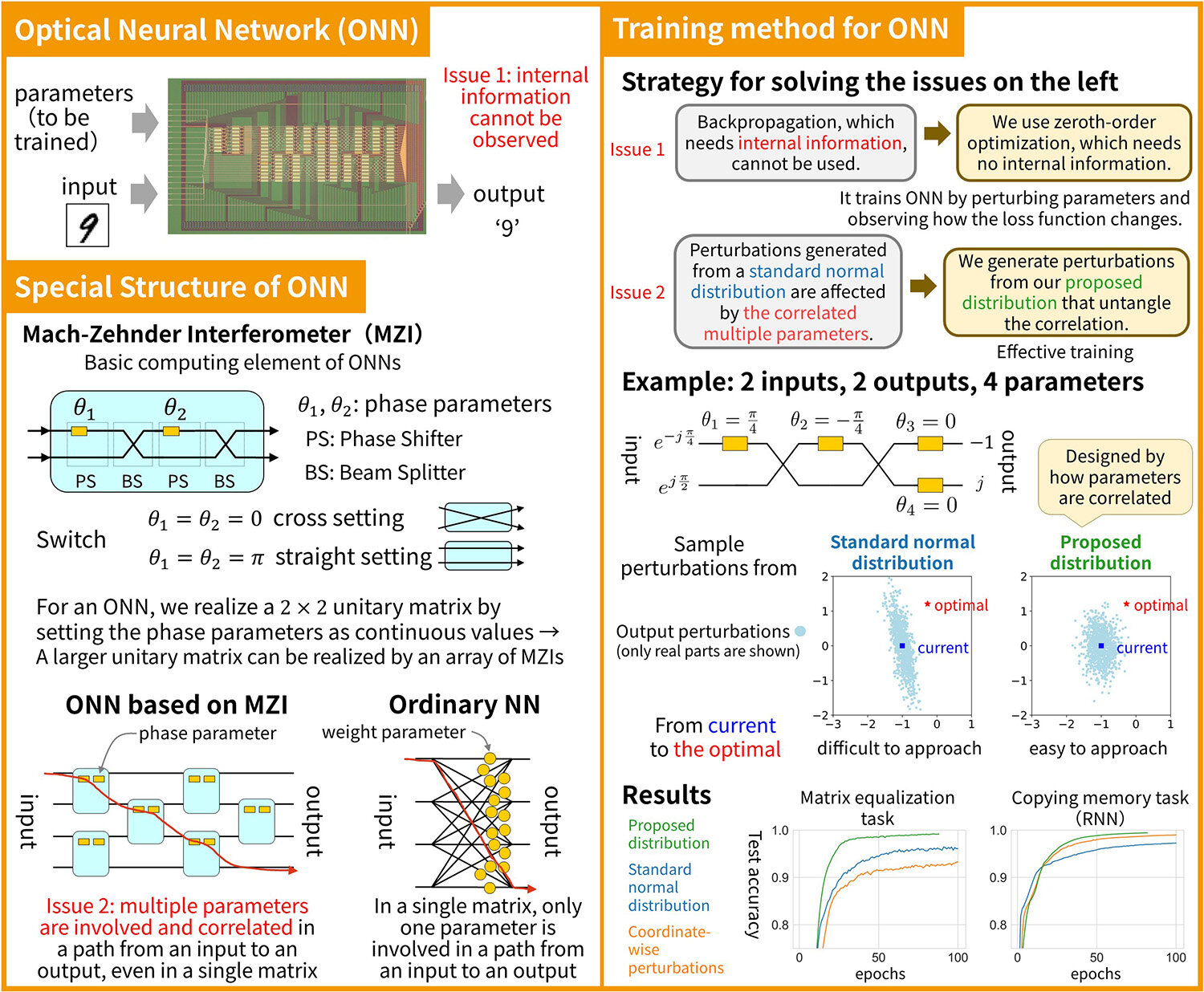
[1] H. Sawada, K. Aoyama, M. Notomi, “Layered-parameter perturbation for zeroth-order optimization of optical neural networks,” in Proc. The 39th AAAI Conference on Artificial Intelligence (AAAI-25), 2025.
Hiroshi Sawada, Learning and Intelligent Systems Research Group, Innovative Communication Laboratory