02 |
Accurate spatial prediction with limited dataMeta-learning based on neural Gaussian processes ![]() |
---|
To improve the prediction accuracy of spatial data using machine learning, a sufficient amount of data are typically required. In this research, we propose a new meta-learning method that learns how to learn from diverse sensor data collected across multiple regions. Our method enables improved prediction even when only a small amount of data are available for new regions and sensors. For effective meta-learning, we introduce a neural Gaussian process model, which combines deep learning - capable of handling complex data - with Gaussian processes, which allow efficient learning from limited data. Our approach can be applied to tasks such as estimating air pollution conditions from a small number of observation points or predicting traffic volume from short-term data. We will further advance meta-learning techniques to build accurate and reliable AI systems, even in data-scarce environments, thereby expanding the range of applications where AI can be effectively utilized.
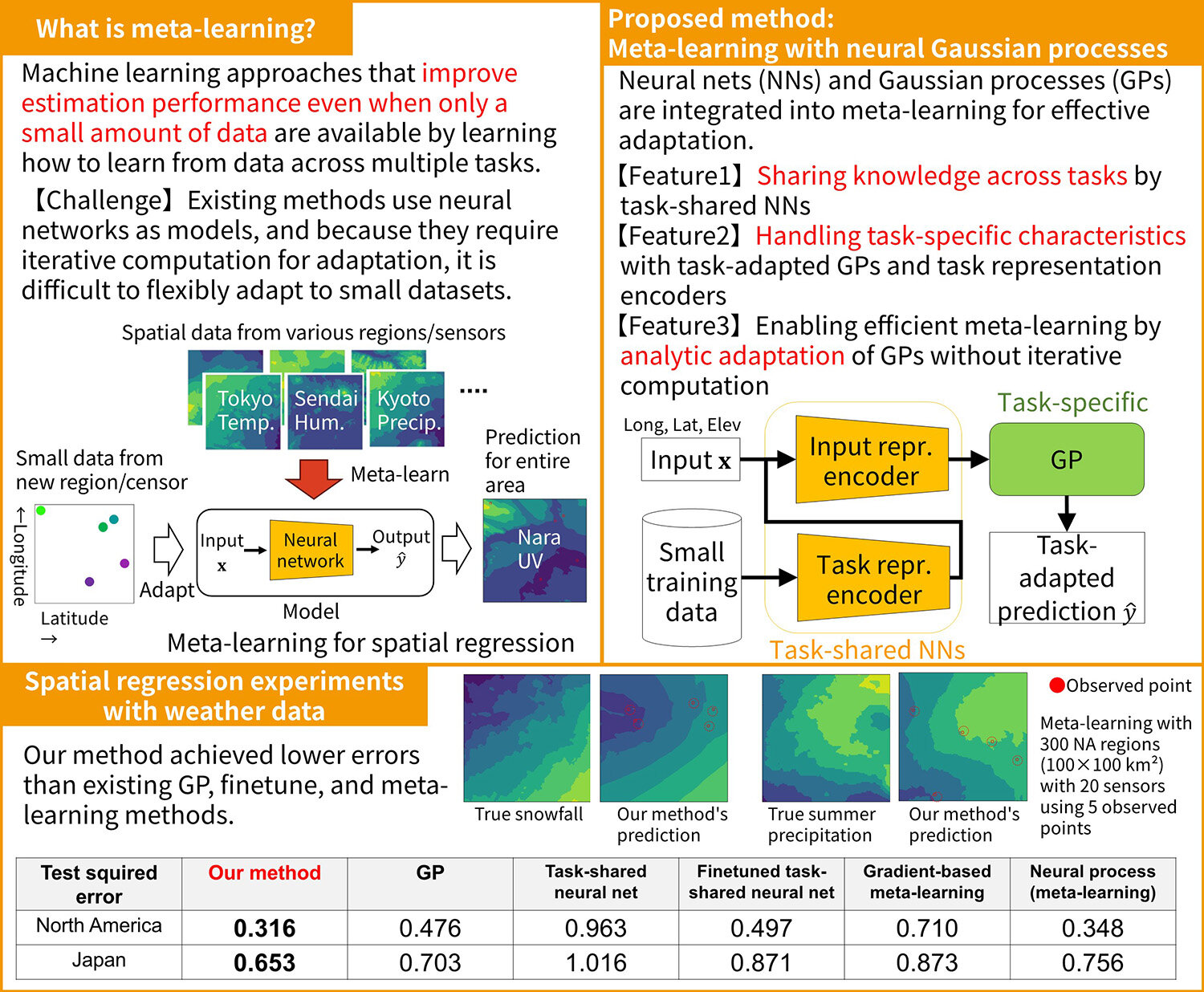
[1] T. Iwata, Y. Tanaka, “Few-shot learning for spatial regression via neural embedding-based Gaussian processes,” Machine Learning, Vol. 111, p. 1239-1257, 2022.
[2] T. Iwata, Y. Tanaka, “Symplectic neural Gaussian processes for meta-learning Hamiltonian dynamics,” in Proc. The 33rd International Joint Conference on Artificial Intelligence (IJCAI), 2024.
[3] T. Iwata, A. Kumagai, “Meta-learning to calibrate Gaussian processes with deep kernels for regression uncertainty estimation,” Neurocomputing, Vol. 579, 2024
Tomoharu Iwata, Learning and Intelligent Systems Research Group, Innovative Communication Laboratory