03 |
AI can adapt to new environments without labelsTheoretical understanding of source-free domain adaptation ![]() |
---|
Deploying AI models in a new environment requires significant costs related to the collection of labeled data for model re-training. Source-free domain adaptation (SFDA) addresses this issue by adapting the model to a new environment using only unlabeled data from the target environment. On the basis of the theory of self-training, we show a unified theoretical understanding of the existing SFDA methods that were individually developed. We also build an improved SFDA method based on two useful insights derived from this theoretical understanding and achieve consistent performance improvements over conventional methods under various conditions. SFDA is attracting significant attention due to its practical utility in that it enables flexible adaptation of the model to new environments while preserving the privacy and copyright of the source training data. Our theoretical understanding of SFDA will improve the reliability of SFDA techniques and serve as a foundation for the creation of new SFDA methods.
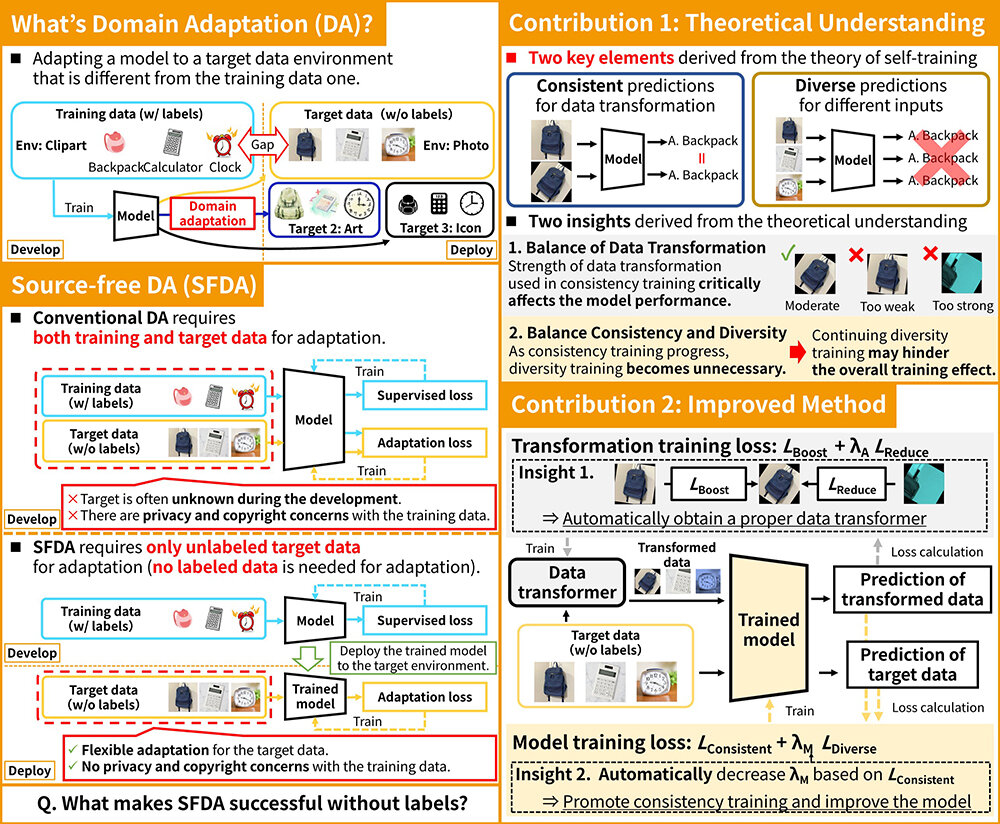
[1] Y. Mitsuzumi, A. Kimura, H. Kashima, “Understanding and improving source-free domain adaptation from a theoretical perspective,” in Proc. The IEEE/CVF Conference on Computer Vision and Pattern Recognition (CVPR), pp. 28515-28524, 2024.
Yu Mitsuzumi, Recognition Research Group, Media Information Laboratory